The Science of Analyzing Social Media Data for Research
In today’s digital age, social media has become a treasure trove of data, waiting to be explored and analyzed. The sheer volume of user-generated content, interactions, and metadata available on platforms like Twitter, Facebook, and Instagram offers researchers unparalleled opportunities to gain insights into societal trends, behaviors, and sentiments. But what exactly does it mean to analyze social media data? It's not just about crunching numbers or counting likes; it's about understanding the intricate web of human interaction that these platforms represent. This article delves into the methodologies, tools, and implications of analyzing social media data, shedding light on its significance across various research fields.
To effectively analyze social media data, one must first grasp its structure and types. Social media data can be broadly categorized into three main types: user-generated content, interactions, and metadata. User-generated content includes posts, comments, and images shared by users, while interactions refer to the likes, shares, and replies that these posts generate. Metadata, on the other hand, provides context about the data, such as timestamps, geolocation, and user demographics. Understanding these components is crucial for researchers as they sift through the vast ocean of information to extract meaningful insights.
When it comes to collecting social media data, researchers have several methods at their disposal. Some of the most common techniques include:
- APIs: Many social media platforms offer Application Programming Interfaces (APIs) that allow researchers to access data in a structured format. This method is often favored for its reliability and ease of use.
- Web Scraping: This technique involves extracting data directly from web pages. While it can capture a wide range of information, it may also raise ethical concerns regarding data ownership and usage.
- Surveys: Researchers may also gather data through surveys, asking users about their social media habits and experiences. This method can provide valuable qualitative insights but may be limited by response bias.
Each of these methods has its own set of advantages and challenges, influencing the overall quality and reliability of the research findings. For instance, while APIs provide structured data, they may come with restrictions on the amount of data that can be collected. On the other hand, web scraping can yield a wealth of information but may require advanced technical skills and raise legal issues. Hence, choosing the right method is a critical step in the research process.
Once the data is collected, the next step is analysis. Researchers employ various techniques to uncover patterns and trends within the data. Some prominent methods include:
- Sentiment Analysis: This technique involves assessing the emotions expressed in social media posts, helping researchers understand public opinion on specific topics.
- Network Analysis: By examining the relationships and interactions between users, researchers can identify influencers and the spread of information.
- Content Analysis: This method focuses on the themes and messages conveyed in social media content, providing insights into cultural trends and societal issues.
Each of these techniques plays a vital role in revealing the underlying narratives within social media data, enabling researchers to draw informed conclusions that can influence policy, marketing strategies, and societal understanding.
As with any research involving human data, ethical considerations are paramount. Issues such as privacy concerns, informed consent, and data ownership must be addressed to conduct responsible research. Researchers need to ensure that they respect user privacy and obtain consent where necessary. Additionally, understanding who owns the data and how it can be used is essential to maintaining public trust. By navigating these ethical challenges, researchers can contribute to a more responsible and trustworthy landscape in social media research.
The analysis of social media data significantly influences various research fields, including sociology, marketing, and political science. For instance, sociologists can gauge public sentiment on social issues, marketers can tailor their strategies based on consumer behavior, and political scientists can analyze voter sentiment during elections. Understanding the impact of social media data analysis on these fields allows researchers to tailor their methodologies to meet specific disciplinary needs, enhancing the relevance and applicability of their findings.
Despite its potential, analyzing social media data is fraught with challenges. Researchers often face issues like data overload, where the sheer volume of information can be overwhelming. Additionally, the prevalence of misinformation on social media can complicate analysis, as distinguishing between credible information and falsehoods becomes increasingly difficult. Furthermore, algorithm biases can skew results, leading to inaccurate conclusions. Recognizing these obstacles is vital for improving research accuracy and reliability.
To navigate the complexities of social media data analysis, researchers have access to a variety of tools and software. These range from basic analytics platforms, which offer insights into engagement metrics, to advanced machine learning applications that can perform complex analyses. Some popular tools include:
- Hootsuite: A user-friendly platform for managing and analyzing social media presence.
- Brandwatch: An advanced analytics tool that provides deep insights into brand perception and customer sentiment.
- NodeXL: A powerful tool for network analysis, helping researchers visualize social media connections.
These tools enhance researchers' ability to process and interpret data effectively, ultimately leading to more robust research outcomes.
Examining case studies of successful social media data analysis provides valuable insights into best practices and innovative methodologies. For example, researchers analyzing Twitter data during a political election were able to predict voter behavior with surprising accuracy by employing sentiment analysis and network analysis techniques. These real-world applications illustrate how social media research can inform decisions and strategies across various sectors.
Looking ahead, the future of social media research is likely to be shaped by advancements in technology, evolving user behaviors, and emerging ethical standards. With the rise of artificial intelligence and machine learning, researchers can expect more sophisticated analysis methods that can handle larger datasets and extract deeper insights. Additionally, as users become more aware of privacy issues, ethical standards in research will need to evolve to maintain trust and transparency. Anticipating these trends will help researchers stay ahead in their analyses and ensure their work remains relevant in an ever-changing digital landscape.
Q: What is social media data analysis?
A: Social media data analysis involves collecting and analyzing user-generated content and interactions on social media platforms to gain insights into public sentiment, trends, and behaviors.
Q: What are the main challenges in analyzing social media data?
A: Key challenges include data overload, misinformation, and algorithm biases that can affect the accuracy and reliability of research findings.
Q: How can ethical concerns be addressed in social media research?
A: Researchers can address ethical concerns by ensuring user privacy, obtaining informed consent, and being transparent about data ownership and usage.

[Understanding Social Media Data]
Social media data is a treasure trove of insights, comprising a vast array of user-generated content, interactions, and metadata. It's like a digital diary where millions of people share their thoughts, experiences, and opinions every single day. To truly understand this data, one must first grasp its structure and the various types it encompasses. From tweets and posts to comments and shares, each piece of data tells a story, and when pieced together, they create a captivating narrative about human behavior.
At its core, social media data can be categorized into several types:
- User-generated content: This includes everything from status updates and photos to videos and articles shared by users.
- Interactions: Likes, shares, comments, and retweets that show how users engage with content.
- Metadata: Information about the content, such as timestamps, geolocation, and device used, which adds context to the data.
Understanding these components is crucial for effective analysis. For instance, analyzing user-generated content can reveal trends in public opinion, while interactions can help gauge the popularity of specific topics. Metadata, on the other hand, can provide insights into when and where discussions are happening, helping researchers to identify patterns over time.
Moreover, the sheer volume of social media data can be overwhelming. Every second, thousands of posts are created across various platforms, generating a flood of information that can either be a boon or a bane for researchers. It's essential to develop a robust framework for filtering and categorizing this data to extract meaningful insights without getting lost in the noise.
As we delve deeper into the analysis of social media data, we must also consider the implications of our findings. The insights gained from this data can influence a wide range of fields, from marketing strategies to public policy decisions. Therefore, understanding social media data isn't just about numbers and trends; it's about interpreting these findings in a way that can drive change and foster understanding in our society.

[Data Collection Methods]
When it comes to analyzing social media data, the first step is collecting that data effectively. There are several methods researchers can use to gather this vast pool of information, each with its own set of advantages and challenges. Understanding these methods is essential for anyone looking to derive meaningful insights from social media platforms.
One of the most popular methods for data collection is through Application Programming Interfaces (APIs). APIs allow researchers to access data directly from social media platforms like Twitter, Facebook, and Instagram. This method is often favored for its reliability and the structured format in which data is provided. However, there are limitations, such as rate limits on the amount of data that can be collected at a time, and restrictions on the types of data available. For instance, while you can gather public posts and interactions, private messages and user information are typically off-limits, raising questions about the completeness of the data.
Another common technique is web scraping. This involves using scripts to extract data from web pages directly. It can be a powerful tool for collecting data that may not be readily available through APIs. However, web scraping comes with its own set of challenges, such as the need to constantly update scripts to adapt to changes in website structures and the potential for violating terms of service. Furthermore, web scraping can lead to data that is less structured and harder to analyze compared to data collected through APIs.
Surveys are also a method worth mentioning. By directly asking users about their behaviors, opinions, and interactions on social media, researchers can gather qualitative data that provides context to the quantitative data collected from APIs and web scraping. However, surveys can suffer from response bias, as individuals may not always provide honest or accurate answers. Additionally, the reach of surveys can be limited, especially if they are not well-promoted.
To summarize, the three primary methods of data collection in social media research include:
- APIs: Structured and reliable data access, but with limitations.
- Web Scraping: Flexible data collection, but requires technical know-how and may violate terms of service.
- Surveys: Direct user input for qualitative insights, but can be biased and limited in reach.
Ultimately, the choice of method depends on the research objectives, the type of data needed, and the resources available. Researchers often find themselves combining these methods to leverage the strengths of each while mitigating their weaknesses. For example, they might use APIs to gather a large dataset, then follow up with surveys to gain deeper insights into specific trends observed in the data.
As the landscape of social media continues to evolve, so too will the methods for collecting data. Staying informed about new tools and techniques is crucial for researchers who want to remain relevant in this fast-paced field.

[Data Analysis Techniques]
Analyzing social media data is like peeling an onion; each layer reveals something new and insightful. The techniques used in this analysis are diverse and tailored to uncover the hidden gems within the vast ocean of user-generated content. One of the most popular methods is sentiment analysis, which involves assessing the emotional tone behind words. This technique allows researchers to gauge public opinion on various topics, from political campaigns to product launches. By employing natural language processing (NLP) algorithms, sentiment analysis can classify text as positive, negative, or neutral, providing a clear picture of how people feel about specific subjects.
Another powerful technique is network analysis, which examines the relationships and interactions between users on social media platforms. Imagine a web of connections where each node represents a user and each edge signifies an interaction. By visualizing these connections, researchers can identify influential users, track the spread of information, and understand community dynamics. This method is particularly useful in fields like sociology and marketing, where understanding social structures can lead to more effective strategies.
Content analysis is yet another crucial technique that focuses on the actual messages being shared on social media. Researchers can categorize and quantify content to identify trends, themes, and patterns over time. For instance, a study might analyze the frequency of specific hashtags or keywords related to a political event, allowing researchers to draw conclusions about public discourse. This technique can also help in understanding how narratives evolve, especially during significant events like elections or crises.
To give you a clearer picture, here’s a brief overview of these techniques in a table:
Technique | Description | Applications |
---|---|---|
Sentiment Analysis | Evaluates the emotional tone of text. | Public opinion research, brand monitoring. |
Network Analysis | Explores relationships and interactions among users. | Social structure analysis, influencer identification. |
Content Analysis | Categorizes and quantifies messages shared online. | Trend identification, narrative evolution studies. |
Moreover, researchers often combine these techniques to enhance their findings. For instance, by integrating sentiment analysis with network analysis, one can not only understand how people feel about a topic but also see who is influencing those sentiments. This holistic approach provides a richer understanding of social media dynamics and can lead to more robust conclusions.
In conclusion, the techniques used for analyzing social media data are not just tools; they are lenses through which researchers can view and interpret the complexities of human interaction in the digital age. Each method has its strengths and can be applied in various contexts, making them invaluable for anyone looking to understand the social landscape better.
Q: What is sentiment analysis and how is it used?
A: Sentiment analysis is a technique used to determine the emotional tone behind a body of text. It is commonly used in marketing to gauge consumer opinions about products or brands.
Q: How does network analysis help in social media research?
A: Network analysis helps researchers understand the relationships and interactions among users, identifying key influencers and the flow of information within social networks.
Q: Can content analysis be done manually?
A: Yes, content analysis can be done manually, but it is often more efficient to use software tools that can automate the process, especially when dealing with large datasets.

[Ethical Considerations]
When diving into the vast ocean of social media data, ethical considerations emerge as critical guiding principles. As researchers, it is our responsibility to navigate these waters with care, ensuring that we respect the rights and privacy of individuals whose data we analyze. One of the foremost ethical concerns is privacy. Social media platforms are filled with personal information, and extracting data without proper consent can lead to significant breaches of privacy. It's essential to ask ourselves: Are we using this data responsibly? Are we infringing on someone's personal space?
Another vital aspect is informed consent. While some data on social media is publicly accessible, the individuals behind that data may not be aware that their posts or interactions are being analyzed for research purposes. Researchers must consider whether they should inform users about how their data will be used and obtain their consent. This practice not only upholds ethical standards but also fosters trust between researchers and the public.
Furthermore, the issue of data ownership cannot be overlooked. Who truly owns the data generated on social media? Is it the users, the platforms, or the researchers who analyze it? This ambiguity can create legal and ethical dilemmas. Researchers must be transparent about their data sources and ensure that they adhere to the terms of service of the platforms they are using. This transparency is crucial for maintaining integrity in research.
To illustrate these ethical considerations, let's take a look at some key principles that can guide researchers in their analysis of social media data:
- Respect for Privacy: Always prioritize the privacy of individuals and anonymize data wherever possible.
- Informed Consent: Seek permission from users when necessary, especially if the data is sensitive.
- Transparency: Be clear about your data sources and how you intend to use the information.
- Accountability: Be prepared to address any ethical concerns that may arise during your research.
In summary, ethical considerations in social media data analysis are not just a box to check; they are fundamental to conducting responsible research. By prioritizing privacy, obtaining informed consent, clarifying data ownership, and adhering to guiding principles, researchers can contribute to a more ethical landscape in the realm of social media research. This approach not only protects individuals but also enhances the credibility and reliability of research findings.
Q1: Why is informed consent important in social media research?
A1: Informed consent is crucial because it respects the autonomy of individuals and ensures that they are aware of how their data will be used, fostering trust between researchers and participants.
Q2: How can researchers protect user privacy while analyzing social media data?
A2: Researchers can protect user privacy by anonymizing data, avoiding the collection of personally identifiable information, and adhering to ethical guidelines that prioritize user confidentiality.
Q3: What are the potential consequences of unethical data analysis?
A3: Unethical data analysis can lead to privacy violations, loss of trust from the public, legal repercussions, and ultimately, the invalidation of research findings.

[Impact on Research Fields]
The impact of social media data analysis on various research fields is nothing short of revolutionary. In today's digital age, platforms like Twitter, Facebook, and Instagram serve as treasure troves of information that can significantly enhance our understanding of human behavior, societal trends, and even political movements. Researchers across disciplines are increasingly leveraging this data to draw insights that were previously inaccessible. But how exactly does this affect different fields? Let’s dive into a few key areas.
In sociology, social media data provides a unique lens through which researchers can observe societal interactions and cultural shifts in real-time. For instance, by analyzing posts and comments, sociologists can identify emerging social norms, track the evolution of public opinion, and even study the dynamics of group behavior. This real-time data can serve as a powerful supplement to traditional research methods, allowing for a more nuanced understanding of societal changes.
Similarly, in the field of marketing, companies are harnessing social media analytics to tailor their strategies and engage with consumers more effectively. By analyzing user-generated content, businesses can gauge sentiment towards their brands, products, and services. This data-driven approach not only helps in crafting targeted marketing campaigns but also enhances customer satisfaction by aligning offerings with consumer preferences. The ability to track trends and measure the impact of marketing efforts in real-time is a game-changer for marketers.
Political science is yet another field transformed by social media data analysis. Researchers can track political sentiments, monitor public reactions to policies, and even predict election outcomes based on social media activity. For example, during election cycles, analyzing hashtags and trending topics can reveal voter sentiment and engagement levels, offering invaluable insights for political strategists and candidates. This data can also help in understanding the spread of misinformation and its effects on public opinion.
To illustrate the profound impact of social media data across research fields, consider the following table:
Research Field | Impact of Social Media Data |
---|---|
Sociology | Real-time observation of social norms and public opinion trends. |
Marketing | Enhanced consumer engagement and targeted marketing strategies. |
Political Science | Insights into voter sentiment and election predictions. |
Moreover, the implications of analyzing social media data extend beyond these fields. In public health, for instance, researchers can monitor the spread of diseases and public health campaigns by analyzing social media conversations. This allows for timely interventions and better resource allocation, ultimately improving health outcomes. The interconnectedness of social media data with various research domains highlights its versatility and importance.
In conclusion, the impact of social media data analysis on research fields is profound and multifaceted. As researchers continue to explore innovative methodologies and tools, the potential for groundbreaking discoveries only grows. By embracing the wealth of information available through social media, we can gain a deeper understanding of the complexities of human behavior and societal dynamics.
- What is social media data analysis? Social media data analysis involves examining user-generated content, interactions, and metadata from social media platforms to extract meaningful insights.
- How does social media data impact research? It provides real-time data that enhances understanding of social trends, consumer behavior, and political sentiments across various fields.
- What are some ethical concerns in social media data analysis? Privacy issues, informed consent, and data ownership are significant ethical considerations that researchers must address.

[Challenges in Data Analysis]
Analyzing social media data is undoubtedly a fascinating endeavor, but it comes with its own set of challenges that can leave even the most seasoned researchers scratching their heads. One of the **most significant hurdles** is the sheer volume of data generated daily across various platforms. Can you imagine trying to sift through millions of tweets, posts, and comments just to find a few relevant insights? It’s like searching for a needle in a haystack, and without the right tools and strategies, this task can quickly become overwhelming.
Another major challenge is the prevalence of **misinformation**. In the age of viral content, false information spreads faster than the truth. Researchers must navigate through this murky water, distinguishing between genuine user sentiments and those influenced by misleading narratives. This challenge not only complicates data interpretation but can also skew research findings, leading to potentially harmful conclusions.
Moreover, there are **algorithmic biases** at play. Social media platforms use complex algorithms to determine what content is shown to users, which can create a skewed representation of public opinion. For instance, if a particular demographic is more likely to engage with certain types of content, researchers may inadvertently miss out on diverse perspectives. This issue is compounded by the fact that different platforms have different user bases and engagement patterns, making it difficult to generalize findings across the board.
In addition to these challenges, researchers must also grapple with **data privacy concerns**. As social media users become more aware of their digital footprints, the ethical implications of data collection and analysis come to the forefront. Questions about informed consent and data ownership are critical. Are researchers using data responsibly? Are they respecting user privacy? These are not just ethical dilemmas; they are legal ones that can have serious repercussions for research projects.
Lastly, the **dynamic nature of social media** presents its own set of challenges. Trends can change overnight, and what is relevant today may be outdated tomorrow. This rapid evolution requires researchers to be agile and adaptable, constantly updating their methodologies to keep pace with the shifting landscape of social media. It’s a bit like trying to catch a moving target; the moment you think you’ve got it in your sights, it shifts again.
To sum it up, the challenges in analyzing social media data are multifaceted and complex. From data overload and misinformation to ethical considerations and algorithmic biases, researchers must navigate a tricky terrain. However, with the right approach and tools, these obstacles can be managed, paving the way for insightful and impactful research.
- What are the main challenges in social media data analysis? The main challenges include data overload, misinformation, algorithmic biases, data privacy concerns, and the dynamic nature of social media.
- How can researchers mitigate misinformation in their studies? Researchers can cross-verify data with multiple sources, use fact-checking tools, and apply sentiment analysis to gauge the authenticity of information.
- Why is data privacy important in social media research? Data privacy is crucial to protect users' rights and maintain trust. Researchers must ensure they have informed consent and respect data ownership.
- What tools can help manage data overload? Tools such as data visualization software, machine learning algorithms, and efficient data management systems can help researchers handle large datasets more effectively.

[Tools for Social Media Analysis]
In the rapidly evolving landscape of social media, having the right tools at your disposal can make all the difference in how effectively you analyze data. With a plethora of options available, researchers and marketers alike need to choose tools that not only fit their specific needs but also enhance their ability to derive meaningful insights from the vast ocean of social media content. From simple analytics platforms to sophisticated machine learning applications, the tools available today cater to a variety of analytical requirements.
One of the most popular tools in social media analysis is Hootsuite. It allows users to manage multiple social media accounts from a single dashboard, track engagement metrics, and analyze audience demographics. Hootsuite’s analytics features enable users to create custom reports that can help identify trends and measure the effectiveness of social media campaigns. However, while it offers a user-friendly interface, the depth of analysis might not satisfy more advanced researchers looking for intricate data.
On the other hand, for those seeking deeper insights, Tableau stands out as a powerful data visualization tool. It allows users to create interactive and shareable dashboards that can illustrate trends and patterns in social media data. With its ability to connect to various data sources, Tableau can handle large datasets, making it a favorite among data scientists and researchers who need to visualize complex information. The learning curve may be steeper than other tools, but the payoff in terms of insight can be substantial.
Another noteworthy tool is Brandwatch, which specializes in social listening and sentiment analysis. It enables researchers to monitor conversations across different platforms and gauge public sentiment around specific topics or brands. By harnessing the power of artificial intelligence, Brandwatch can analyze the tone of conversations, helping researchers understand not just what people are saying, but how they feel about it. This capability is invaluable for fields like marketing and political science, where understanding public perception is crucial.
For those who prefer a more hands-on approach, Python libraries such as Tweepy and Beautiful Soup are excellent for custom data scraping and analysis. These tools allow researchers to pull data directly from social media platforms and perform tailored analyses based on their specific research questions. While this method requires a certain level of programming knowledge, the flexibility and customization it offers can lead to highly relevant findings.
To provide a clearer picture of the tools available, here’s a comparative table highlighting some key features:
Tool | Best For | Key Features |
---|---|---|
Hootsuite | Social Media Management | Multi-account management, engagement tracking, custom reporting |
Tableau | Data Visualization | Interactive dashboards, large dataset handling, data source integration |
Brandwatch | Social Listening | Sentiment analysis, conversation monitoring, trend identification |
Python Libraries | Custom Analysis | Data scraping, tailored data processing, flexibility |
Ultimately, the choice of tools depends on the specific goals of the research. Whether you are looking to manage your social media presence, visualize complex datasets, or dive deep into sentiment analysis, there is a tool out there that can help you achieve your objectives. The key is to remain adaptable and willing to explore different options to find the perfect fit for your research needs.
- What is social media analysis? Social media analysis involves examining user-generated content, interactions, and metadata on social platforms to derive insights about trends, sentiments, and behaviors.
- Why is it important to analyze social media data? Analyzing social media data helps researchers and businesses understand public opinion, track brand performance, and identify emerging trends.
- What are some common tools used for social media analysis? Common tools include Hootsuite, Tableau, Brandwatch, and various Python libraries for custom analysis.
- How can I ensure ethical practices in social media analysis? Researchers should prioritize user privacy, obtain informed consent when necessary, and be transparent about data usage.
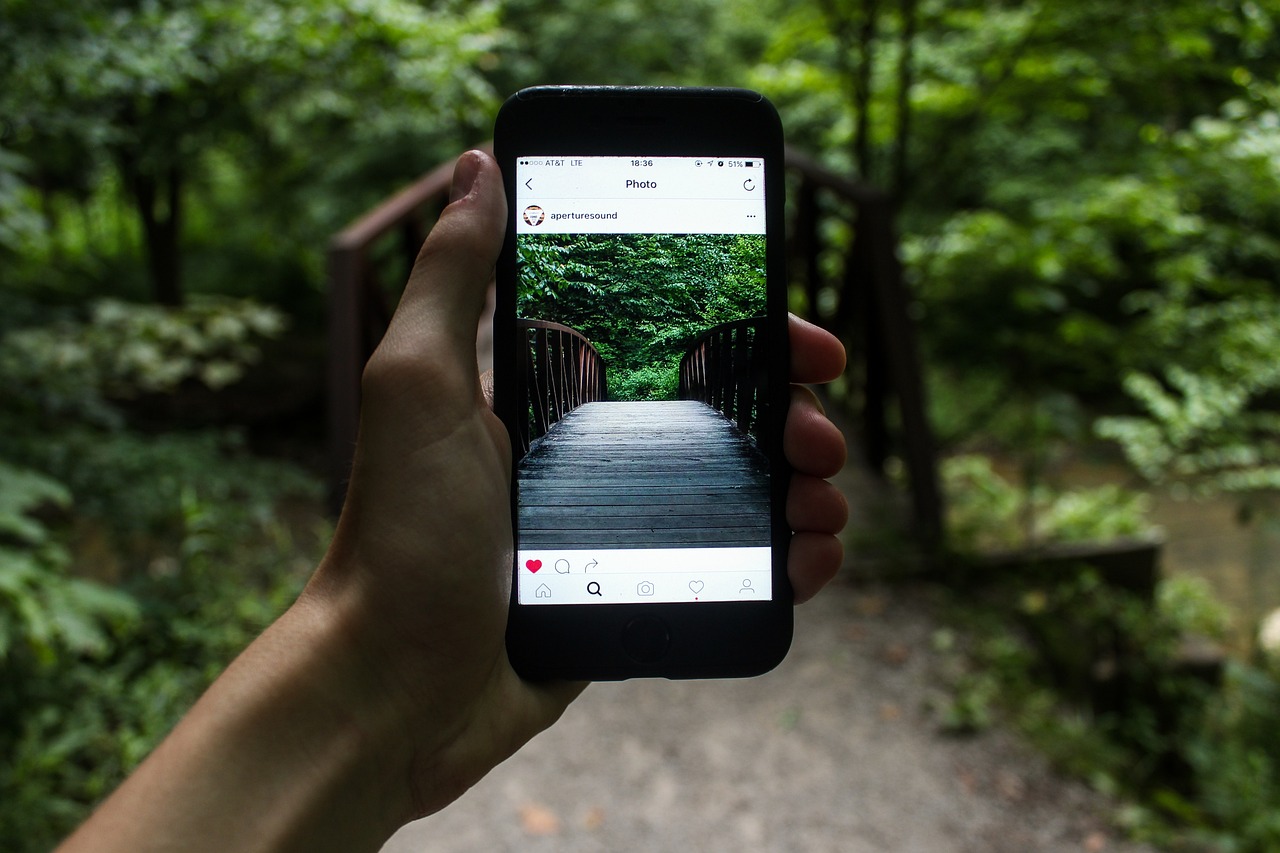
[Case Studies]
When it comes to analyzing social media data, real-life case studies can provide invaluable lessons and insights. These studies illustrate how researchers have successfully navigated the complexities of social media data analysis, leading to impactful findings that resonate across various fields. For instance, consider the case of a marketing firm that utilized social media data to understand consumer behavior during a product launch. By employing sentiment analysis on tweets and posts related to their brand, they were able to gauge public opinion in real-time. This allowed them to tweak their marketing strategies on the fly, ultimately leading to a 30% increase in sales compared to previous launches.
Another fascinating example comes from the field of sociology. Researchers studying the impact of social media on community engagement analyzed posts from a local Facebook group. They discovered that interactions within the group were not just about sharing information but also about fostering a sense of belonging among members. This finding emphasized the role of social media as a platform for community building, rather than just a tool for communication. By utilizing network analysis, the researchers mapped out the connections between users and identified key influencers who played pivotal roles in driving discussions.
These case studies underscore the significance of choosing the right methodologies for specific research objectives. In some instances, qualitative approaches, such as content analysis, may be more suitable than quantitative methods. For example, a study focusing on social movements on Twitter might benefit from a qualitative examination of hashtags and narratives, revealing deeper meanings behind the data. This approach allows researchers to capture the emotional undertones and collective sentiments that numbers alone might miss.
To provide a clearer understanding, here’s a summary of key findings from notable case studies:
Field | Case Study Focus | Findings |
---|---|---|
Marketing | Product Launch | 30% increase in sales through sentiment analysis |
Sociology | Community Engagement | Social media fosters a sense of belonging |
Political Science | Election Campaigns | Influencer impact on voter sentiments |
In conclusion, these case studies not only highlight the diverse applications of social media data analysis but also emphasize the importance of adapting methodologies to the unique challenges and questions posed by each research endeavor. As the landscape of social media continues to evolve, so too will the strategies researchers employ, making it crucial to stay informed about the latest trends and best practices.
- What is social media data analysis? Social media data analysis involves examining user-generated content and interactions on platforms like Twitter, Facebook, and Instagram to derive insights about behaviors, trends, and sentiments.
- Why are case studies important in social media research? Case studies provide real-world examples of how methodologies can be applied effectively, demonstrating the practical implications of research findings.
- What are some common challenges in social media data analysis? Common challenges include data overload, misinformation, and issues related to privacy and ethics.

[Future Trends in Social Media Research]
The landscape of social media research is constantly evolving, shaped by rapid technological advancements and changing user behaviors. As we look to the future, several trends are emerging that will significantly influence how researchers approach social media data analysis. One of the most notable trends is the increasing use of artificial intelligence (AI) and machine learning algorithms. These technologies are becoming indispensable tools for processing vast amounts of social media data, enabling researchers to uncover patterns and insights that were previously unattainable. For instance, AI can automate the tedious task of data collection and analysis, allowing researchers to focus on interpreting results and making informed decisions.
Another trend to watch is the growing emphasis on data privacy and ethical standards. As users become more aware of their digital footprints and the implications of their online activities, researchers must navigate the delicate balance between obtaining valuable insights and respecting individual privacy. This has led to the development of stricter guidelines and ethical frameworks that govern how social media data is collected and analyzed.
Moreover, the rise of multimedia content on social media platforms—such as videos, images, and live streams—presents both challenges and opportunities for researchers. Traditional text-based analysis methods may not suffice in capturing the richness of these new formats. Thus, researchers will need to develop innovative methodologies that incorporate visual and audio data analysis to gain a comprehensive understanding of social media dynamics.
Furthermore, as social media platforms continue to evolve, researchers will need to adapt their strategies to account for changes in user behavior. For example, the increasing popularity of ephemeral content—like stories on Instagram and Snapchat—requires a shift in how data is collected and analyzed. Researchers must be agile, continuously updating their approaches to stay relevant in this fast-paced digital environment.
In addition, the integration of cross-platform analysis is likely to become more prevalent. Social media users often engage with multiple platforms, and understanding these interactions can provide deeper insights into user behavior. By analyzing data across various platforms, researchers can paint a more holistic picture of social media influence on society.
Lastly, as we move forward, the importance of interdisciplinary collaboration will continue to rise. Social media research intersects with numerous fields, including psychology, sociology, marketing, and political science. By fostering collaboration among experts from diverse backgrounds, researchers can develop more robust methodologies and gain richer insights into the implications of social media on various aspects of life.
In conclusion, the future of social media research is poised to be shaped by technological advancements, ethical considerations, and evolving user behaviors. By staying ahead of these trends, researchers can enhance their methodologies and contribute meaningfully to our understanding of societal dynamics in the digital age.
- What role does AI play in social media research?
AI helps automate data collection and analysis, allowing researchers to uncover insights more efficiently. - How important is data privacy in social media research?
Data privacy is crucial, as researchers must balance obtaining insights with respecting user privacy and adhering to ethical standards. - What challenges do researchers face with multimedia content?
Researchers need to develop new methodologies to analyze visual and audio data effectively, as traditional text-based methods may not suffice.
Frequently Asked Questions
- What is social media data?
Social media data refers to the vast amounts of user-generated content, interactions, and metadata produced on platforms like Facebook, Twitter, and Instagram. This data includes posts, comments, likes, shares, and even the profiles of users, which researchers analyze to gain insights into societal trends and behaviors.
- How is social media data collected?
There are several methods for collecting social media data, including APIs (Application Programming Interfaces), web scraping, and surveys. Each method offers its own set of advantages and challenges, such as ease of access, data quality, and ethical considerations, which can significantly impact the reliability of research findings.
- What are the common data analysis techniques used?
Researchers use various data analysis techniques to interpret social media data, including sentiment analysis, which gauges public opinion; network analysis, which explores relationships between users; and content analysis, which examines the themes and topics present in social media posts. These methods help uncover patterns and trends that inform research.
- What ethical considerations should researchers be aware of?
When analyzing social media data, researchers must navigate several ethical concerns, including privacy issues, the need for informed consent, and questions of data ownership. Addressing these challenges is crucial to conducting responsible research that maintains public trust and adheres to ethical standards.
- How does social media data impact various research fields?
Social media data analysis has a significant influence on fields such as sociology, marketing, and political science. By understanding how social media shapes public opinion and behavior, researchers can tailor their methodologies to better suit the specific needs and challenges of their respective disciplines.
- What challenges do researchers face in social media data analysis?
Researchers encounter numerous challenges when analyzing social media data, including data overload, the prevalence of misinformation, and algorithmic biases. Recognizing these obstacles is essential for improving the accuracy and reliability of research outcomes.
- What tools are available for social media analysis?
A wide array of tools and software solutions exist for social media data analysis, ranging from basic analytics platforms to advanced machine learning applications. These tools assist researchers in effectively processing and interpreting large datasets, enhancing their ability to draw meaningful conclusions.
- Can you provide examples of successful social media data analysis?
Examining case studies of successful social media data analysis can offer valuable insights into best practices and innovative methodologies. These examples showcase how research findings can be applied in real-world scenarios, demonstrating the practical applications of social media analysis.
- What are the future trends in social media research?
The future of social media research is expected to be shaped by advancements in technology, changes in user behavior, and evolving ethical standards. By anticipating these trends, researchers can stay ahead in their analyses and continue to provide relevant insights into the ever-changing landscape of social media.