How Artificial Intelligence is Changing Decision-Making
In today's fast-paced world, the way we make decisions is undergoing a seismic shift, thanks to the integration of Artificial Intelligence (AI). Imagine having a super-smart assistant that can analyze vast amounts of data in seconds, uncover patterns, and provide insights that were previously unimaginable. That's precisely what AI is doing across various sectors, transforming decision-making processes into something more efficient and effective. Whether you're a business leader, a healthcare professional, or simply a curious individual, understanding how AI influences decision-making is essential.
At its core, AI leverages data—lots of it. But not just any data; we're talking about high-quality, relevant information that can drive impactful decisions. The magic happens when AI algorithms sift through this data, learning from it, and making predictions that help us navigate complex choices. For instance, in the business world, AI tools can analyze market trends, customer behaviors, and even competitor strategies to inform strategic decisions. This isn't just about crunching numbers; it's about gaining a competitive edge.
But wait, there's more! The implications of AI in decision-making extend beyond business. In healthcare, AI is revolutionizing how doctors diagnose and treat patients. Imagine a world where AI can quickly analyze medical histories, lab results, and even genetic information to provide tailored treatment plans. This not only enhances the accuracy of diagnoses but also improves patient outcomes, making healthcare more personalized and effective.
However, it's not all rainbows and butterflies. With great power comes great responsibility. As AI systems become more prevalent, we must grapple with ethical considerations surrounding bias, transparency, and accountability. For example, if an AI system is trained on biased data, it can lead to unfair outcomes that disproportionately affect certain groups. Addressing these issues is crucial to ensure that AI-driven decision-making is equitable and just.
Looking ahead, the future of AI in decision-making is brimming with possibilities. As technology continues to advance, we can expect even greater collaboration between humans and AI. Picture a scenario where AI not only assists in decision-making but also empowers individuals to make more informed choices, enhancing our overall quality of life.
In conclusion, the transformative impact of AI on decision-making is profound and far-reaching. As we embrace these changes, it's vital to remain vigilant about the ethical implications and strive for a future where AI serves as a force for good. So, whether you're excited about the prospects or cautious about the challenges, one thing is clear: AI is here to stay, and it's reshaping the way we think about decisions.
- What is AI decision-making?
AI decision-making refers to the process where artificial intelligence systems analyze data and provide insights or recommendations to help humans make informed choices.
- How does AI improve business strategy?
AI enhances business strategy by providing data-driven insights, predictive analytics, and identifying market trends, allowing businesses to make better-informed decisions.
- What are the ethical concerns related to AI?
Ethical concerns include bias in AI algorithms, transparency in decision-making processes, and accountability for AI-driven outcomes.
- How is AI used in healthcare?
AI is used in healthcare to improve diagnostic accuracy, personalize treatment plans, and analyze patient data for better health outcomes.

The Role of Data in AI Decision-Making
Data is the backbone of artificial intelligence (AI) systems, acting as the fuel that powers decision-making processes across various sectors. In today's digital age, the sheer volume of data generated every second is staggering. From social media interactions to online transactions, the data collected is not just vast but also incredibly diverse. This diversity is crucial, as it allows AI systems to learn and adapt in ways that were previously unimaginable. But how does this all work? Well, it starts with the collection, processing, and analysis of data, which collectively influence the quality and effectiveness of AI-driven decisions.
When we talk about data collection, we're referring to the methods and technologies used to gather information from various sources. This can include anything from customer feedback surveys to sensor data from smart devices. The more comprehensive and accurate the data, the better the AI can perform. For instance, consider a retail company that collects data on customer purchasing habits. By analyzing this data, the AI can identify trends and preferences, allowing businesses to tailor their marketing strategies effectively.
Next comes data processing, where raw data is transformed into a format that AI algorithms can understand. This step is crucial because, without proper processing, the data can be misleading or even harmful. Imagine trying to navigate a ship without a map; it would be nearly impossible to reach your destination. Similarly, AI needs well-structured data to make informed decisions. Techniques such as data cleaning and normalization are employed to ensure that the data is accurate and usable.
Once the data is processed, the real magic happens during analysis. AI systems use various algorithms to sift through the data, looking for patterns and insights that humans might overlook. This is where the predictive power of AI shines. For example, in the finance sector, AI can analyze historical market data to predict future stock trends, helping investors make smarter decisions. But it's not just about making predictions; it's also about enhancing the overall decision-making process. AI can provide recommendations based on data-driven insights, helping businesses and individuals make choices that are more likely to lead to positive outcomes.
However, the role of data in AI decision-making is not without challenges. Issues such as data privacy and security are paramount. As organizations collect more data, they must also ensure that they are handling it responsibly. Furthermore, the quality of the data is essential; garbage in, garbage out, as the saying goes. If the data is flawed or biased, the decisions made by AI systems will also be flawed, potentially leading to disastrous consequences.
In summary, the role of data in AI decision-making is multifaceted and critical. It encompasses the entire lifecycle from collection to analysis, influencing every aspect of how AI systems operate. As we continue to advance in technology, the importance of high-quality data will only grow, shaping the future of decision-making in profound ways.
- What types of data are most important for AI decision-making?
Data types vary by industry but typically include structured data (like databases) and unstructured data (like social media posts).
- How do organizations ensure data quality?
Organizations use data cleaning techniques, validation processes, and regular audits to maintain data quality.
- What are the ethical implications of using data in AI?
Ethical implications include concerns about privacy, consent, and the potential for biased outcomes based on flawed data.

AI in Business Strategy
The world of business is evolving at an unprecedented pace, and at the heart of this transformation is artificial intelligence (AI). Companies are no longer making decisions based solely on gut feelings or outdated data; instead, they are embracing data-driven strategies powered by AI. This shift is not just a trend; it’s a revolution that is reshaping how organizations operate and compete in the marketplace.
Imagine being able to predict market trends before they happen or understanding customer needs with pinpoint accuracy. This is the power of AI in business strategy. By leveraging advanced algorithms and machine learning, businesses can analyze vast amounts of data to uncover insights that were previously hidden. This enables organizations to make informed decisions that enhance their competitive advantage. But how exactly does this work?
AI tools can process data from various sources—social media, sales figures, customer feedback, and even market research—to create a comprehensive picture of the business landscape. For instance, companies like Amazon and Netflix utilize AI to analyze user behavior, allowing them to recommend products or shows tailored to individual preferences. This not only boosts customer satisfaction but also drives sales.
Furthermore, AI can assist in predictive analytics, a crucial aspect of business strategy. By examining historical data, AI can forecast future trends and consumer behavior. This means businesses can anticipate changes in demand, optimize inventory levels, and even adjust marketing strategies proactively. The implications are profound: organizations can reduce waste, enhance efficiency, and ultimately increase profitability.
Predictive analytics is like having a crystal ball that helps businesses see into the future. It uses statistical algorithms and machine learning techniques to identify the likelihood of future outcomes based on historical data. For example, a retail company might analyze past sales data to predict which products will be in demand during the upcoming holiday season. This allows them to stock up on popular items and avoid overstocking less desirable products.
To illustrate this, consider the following table that outlines key benefits of predictive analytics in business strategy:
Benefit | Description |
---|---|
Improved Decision-Making | Data-driven insights lead to more informed and strategic decisions. |
Cost Efficiency | Minimizing waste and optimizing resources through accurate forecasting. |
Enhanced Customer Experience | Personalized recommendations and services based on customer data. |
Risk Mitigation | Identifying potential issues before they become significant problems. |
However, while the advantages of predictive analytics are clear, businesses must also navigate several challenges. Issues such as data quality and model accuracy can hinder the effectiveness of AI-driven decisions. Companies need to ensure that the data they collect is accurate, relevant, and up-to-date. Additionally, they must invest in training their teams to interpret AI-generated insights correctly.
In essence, AI is not just a tool; it’s a game-changer in business strategy. It empowers organizations to make decisions backed by data, leading to better outcomes and enhanced efficiency. As businesses continue to integrate AI into their strategies, the potential for growth and innovation is limitless.
- How does AI improve business decision-making?
AI enhances decision-making by providing data-driven insights that allow businesses to predict trends, understand customer behavior, and optimize operations. - What industries are benefiting from AI in business strategy?
Industries such as retail, healthcare, finance, and manufacturing are leveraging AI to improve efficiency, customer experience, and profitability. - What are the challenges of implementing AI in business?
Challenges include data quality issues, the need for skilled personnel, and the potential for bias in AI algorithms. - Can small businesses use AI effectively?
Absolutely! Many AI tools are scalable and affordable, making them accessible for small businesses looking to enhance their decision-making processes.
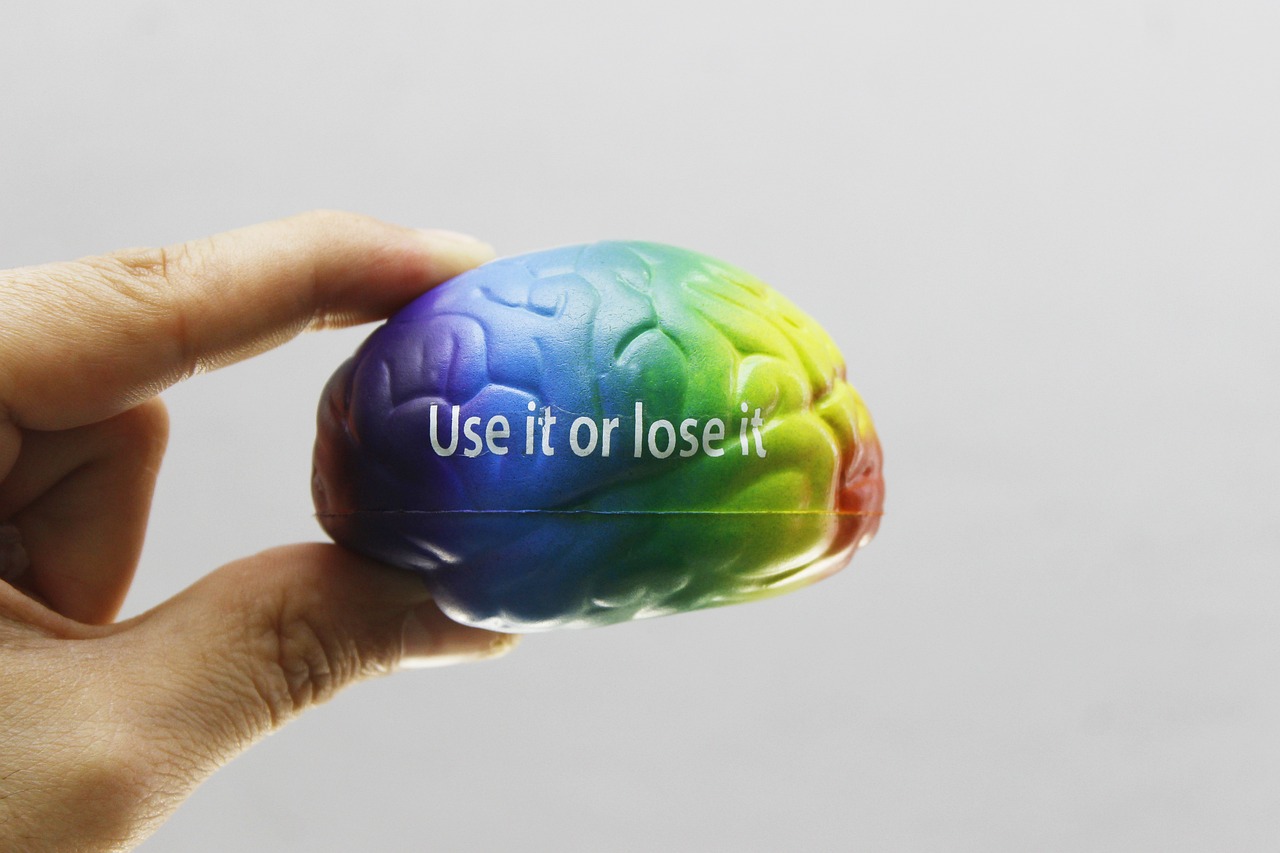
Predictive Analytics and Forecasting
Predictive analytics is like having a crystal ball for businesses, allowing them to gaze into the future using the power of historical data. Imagine being able to anticipate customer behavior, market trends, and even potential pitfalls before they happen. This isn't just wishful thinking; it's a reality made possible by sophisticated algorithms and machine learning techniques. By analyzing patterns and correlations within vast datasets, organizations can make informed decisions that significantly enhance their strategic planning and operational efficiency.
At its core, predictive analytics involves the use of statistical techniques and machine learning to forecast outcomes based on historical data. For instance, retailers can analyze past purchasing behaviors to predict what products will be in demand during upcoming seasons. This not only helps in inventory management but also in tailoring marketing strategies to meet customer expectations. The beauty of predictive analytics lies in its ability to minimize risks and maximize opportunities, making it an invaluable tool for businesses across various sectors.
To put this into perspective, let's consider a few key areas where predictive analytics shines:
- Customer Insights: By analyzing customer data, companies can identify trends and preferences, allowing them to personalize offerings and improve customer satisfaction.
- Operational Efficiency: Businesses can streamline operations by predicting equipment failures or supply chain disruptions, ultimately saving time and resources.
- Financial Forecasting: Predictive models can help in budgeting and financial planning by forecasting revenues and expenses based on historical data.
However, it's essential to understand that predictive analytics isn't foolproof. The accuracy of predictions heavily relies on the quality of data being analyzed. Poor data can lead to misguided decisions, which is why businesses must invest in robust data management practices. Moreover, as the technology evolves, companies must continuously refine their models to adapt to changing market dynamics.
In conclusion, predictive analytics is not just a buzzword; it's a game-changer in decision-making. By leveraging historical data to forecast future trends, businesses can navigate the complexities of their industries with greater confidence. As organizations continue to embrace these advanced analytical tools, the potential for enhanced decision-making and strategic planning will only grow, paving the way for a more data-driven future.
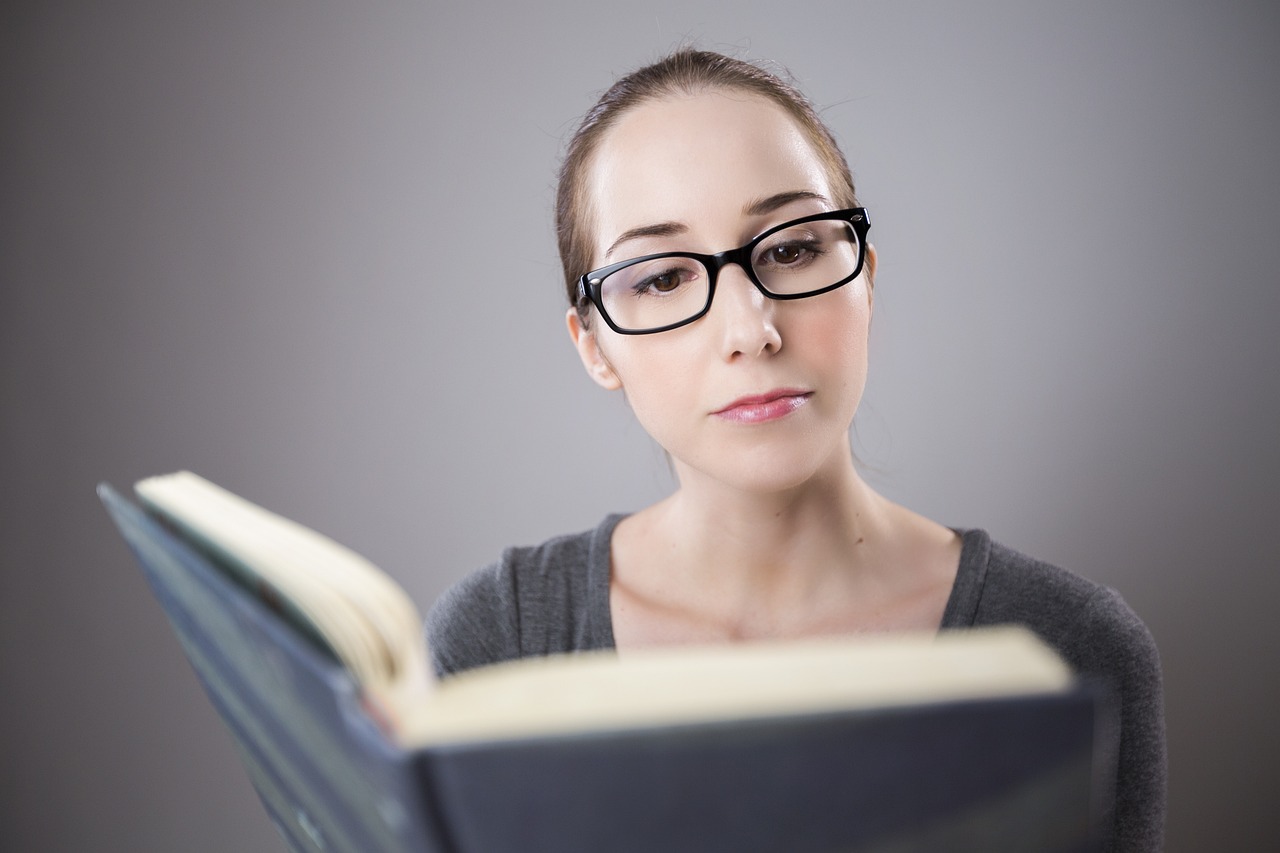
Case Studies of Successful Implementations
When we talk about predictive analytics and its impact on decision-making, real-world examples often provide the most compelling evidence of its effectiveness. Companies across various sectors have embraced AI technologies to enhance their operational efficiencies and drive better outcomes. Let's explore some remarkable case studies that highlight the successful integration of predictive analytics into decision-making processes.
One standout example is Netflix, which has revolutionized how content is delivered and consumed. By leveraging vast amounts of viewer data, Netflix employs predictive analytics to understand viewer preferences and behaviors. This enables them to make informed decisions about content creation and acquisition. For instance, their decision to produce the series "House of Cards" was driven by data insights indicating a strong viewer interest in political dramas. The result? A massive success that not only attracted millions of viewers but also solidified Netflix's position as a leader in original content production.
Another compelling case is that of Amazon. The e-commerce giant uses predictive analytics to enhance customer experiences and optimize inventory management. Through analyzing purchasing patterns, Amazon can predict which products are likely to be in demand, allowing them to stock items accordingly. This not only minimizes overstock and reduces costs but also ensures that customers find what they want when they want it. In fact, Amazon's recommendation engine, which suggests products based on previous purchases, is a direct application of predictive analytics that has significantly increased their sales and customer satisfaction.
In the realm of healthcare, Mount Sinai Health System in New York has implemented predictive analytics to improve patient care and operational efficiency. By analyzing patient data, the hospital can predict which patients are at risk of readmission. This insight allows healthcare providers to intervene proactively, offering tailored support to those patients. As a result, Mount Sinai has seen a substantial decrease in readmission rates, ultimately leading to better patient outcomes and reduced healthcare costs.
These case studies illustrate the transformative power of predictive analytics in decision-making across various industries. Companies that harness the potential of data-driven insights not only gain a competitive edge but also foster innovation and improve overall efficiency.
- What is predictive analytics? Predictive analytics is a branch of advanced analytics that uses historical data, statistical algorithms, and machine learning techniques to identify the likelihood of future outcomes based on historical data.
- How can businesses implement predictive analytics? Businesses can implement predictive analytics by investing in the right tools and technologies, training their staff, and ensuring they have access to quality data.
- What are some challenges faced in predictive analytics? Challenges include data quality issues, the complexity of algorithms, and the need for skilled personnel to interpret data effectively.
- Is predictive analytics applicable to all industries? Yes, predictive analytics can be applied across various industries, including finance, healthcare, retail, and more, to enhance decision-making processes.

Challenges in Predictive Analytics
While predictive analytics offers a treasure trove of insights for businesses, it’s not without its hurdles. One of the most significant challenges is data quality. If the data fed into the system is flawed, outdated, or incomplete, the predictions generated will likely lead to misguided decisions. Imagine trying to navigate a ship with a faulty compass; you may end up far from your intended destination. Therefore, ensuring high-quality data is paramount for successful predictive analytics.
Another challenge is the issue of model accuracy. Predictive models must be rigorously tested and validated to ensure they produce reliable outcomes. A model that performs well on historical data might not necessarily predict future events accurately. This discrepancy can stem from various factors, including changing market conditions or unexpected external influences. Businesses must continuously monitor and adjust their models to maintain their predictive power.
Moreover, there’s the challenge of interpretability. Many predictive analytics tools operate as "black boxes," meaning their internal workings are not transparent. This lack of transparency can lead to difficulties in understanding how decisions are made, which can be particularly problematic in sectors like healthcare or finance where accountability is crucial. Stakeholders need to trust the system, and without clear explanations of how predictions are derived, skepticism can arise.
Additionally, resource constraints can hinder the implementation of predictive analytics. Smaller businesses, in particular, may lack the financial and human resources to invest in sophisticated analytics tools or hire skilled data scientists. This disparity can widen the gap between large corporations that can afford advanced analytics and smaller firms that struggle to keep up.
Lastly, organizations must also navigate the ethical implications of predictive analytics. The potential for biased outcomes is a significant concern. If the data used to train predictive models reflects historical biases, the resulting predictions may perpetuate those biases. Therefore, it’s essential for businesses to implement strategies that identify and mitigate bias within their analytics processes.
In summary, while predictive analytics can dramatically enhance decision-making, it comes with a set of challenges that organizations must address. From ensuring data quality and model accuracy to understanding the ethical implications, businesses need to adopt a comprehensive approach to overcome these obstacles.
- What is predictive analytics? Predictive analytics uses statistical algorithms and machine learning techniques to identify the likelihood of future outcomes based on historical data.
- What are the main challenges of predictive analytics? The main challenges include data quality, model accuracy, interpretability, resource constraints, and ethical implications.
- How can businesses improve data quality? Businesses can improve data quality by implementing robust data management practices, regular audits, and using data cleansing tools.
- Why is model accuracy important? Model accuracy is crucial because inaccurate predictions can lead to poor decision-making, resulting in financial loss or missed opportunities.
- How can organizations mitigate bias in predictive analytics? Organizations can mitigate bias by using diverse datasets, regularly auditing their models, and involving a diverse group of stakeholders in the analytics process.
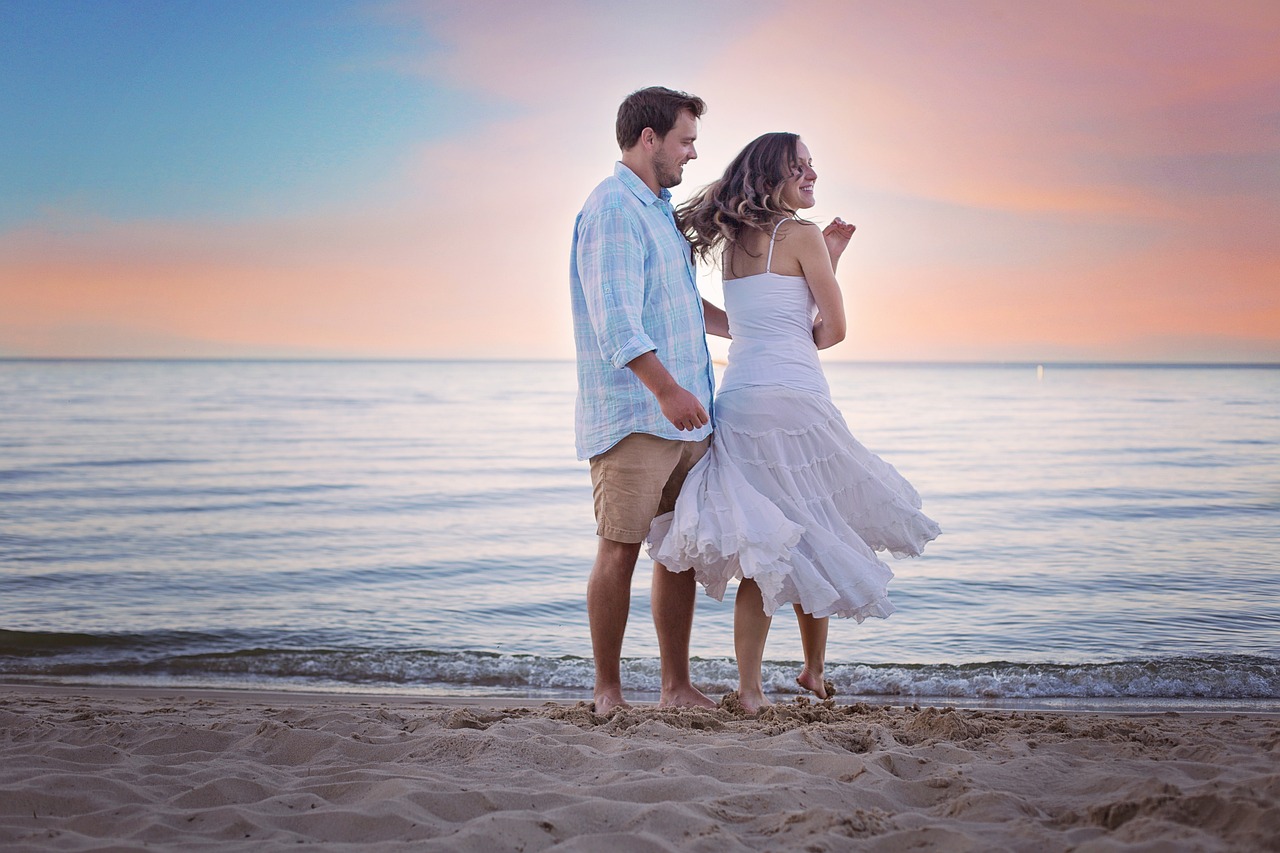
AI in Healthcare Decision-Making
Artificial Intelligence is shaking up the healthcare landscape in ways we could only dream about a few years ago. Imagine walking into a doctor's office where your medical history is instantly analyzed by a sophisticated AI system, providing real-time insights that help your healthcare provider make informed decisions. This isn't science fiction; it's happening right now! AI is revolutionizing healthcare by enhancing diagnostic accuracy, personalizing treatment plans, and improving patient outcomes.
One of the most significant impacts of AI in healthcare is its ability to process vast amounts of data quickly and accurately. From electronic health records (EHRs) to clinical trial data, AI can sift through this information and identify patterns that humans might miss. For instance, AI algorithms can analyze symptoms and medical histories to suggest possible diagnoses, allowing doctors to focus on the most probable conditions. This not only saves time but also reduces the chances of misdiagnosis, which can be life-threatening.
Moreover, AI is playing a pivotal role in treatment planning. By utilizing machine learning algorithms, healthcare providers can create personalized treatment plans tailored to individual patients. This means that instead of a one-size-fits-all approach, patients receive care that considers their unique genetic makeup, lifestyle, and preferences. The result? Improved adherence to treatment and better overall health outcomes.
Let's take a closer look at some specific applications of AI in healthcare decision-making:
- Diagnostic Support: AI tools can assist radiologists in interpreting medical images, such as X-rays and MRIs, by highlighting areas of concern that require further investigation.
- Predictive Analytics: By analyzing historical patient data, AI can predict which patients are at risk of developing certain conditions, allowing for early intervention and preventive measures.
- Clinical Decision Support Systems (CDSS): These AI-driven systems provide healthcare professionals with evidence-based recommendations, enhancing the quality of care delivered to patients.
However, with these advancements come challenges. The integration of AI into healthcare decision-making raises important questions about data privacy, security, and the need for transparency in AI algorithms. Patients must feel confident that their personal health information is being handled responsibly and that AI systems are free from biases that could affect their care.
In conclusion, AI is not just a tool; it’s a game-changer in healthcare decision-making. By enhancing diagnostic accuracy, personalizing treatment plans, and improving patient outcomes, AI is paving the way for a more efficient and effective healthcare system. As we continue to embrace these technologies, it’s crucial to address the ethical considerations that come with them to ensure that AI serves as a force for good in the healthcare industry.
- What are the main benefits of AI in healthcare? AI enhances diagnostic accuracy, personalizes treatment, and improves patient outcomes by analyzing large datasets quickly.
- How does AI help in diagnosing diseases? AI algorithms can analyze symptoms and medical histories to suggest possible diagnoses, reducing the chances of misdiagnosis.
- What ethical concerns are associated with AI in healthcare? Issues such as data privacy, algorithm transparency, and potential biases in AI systems are critical considerations.
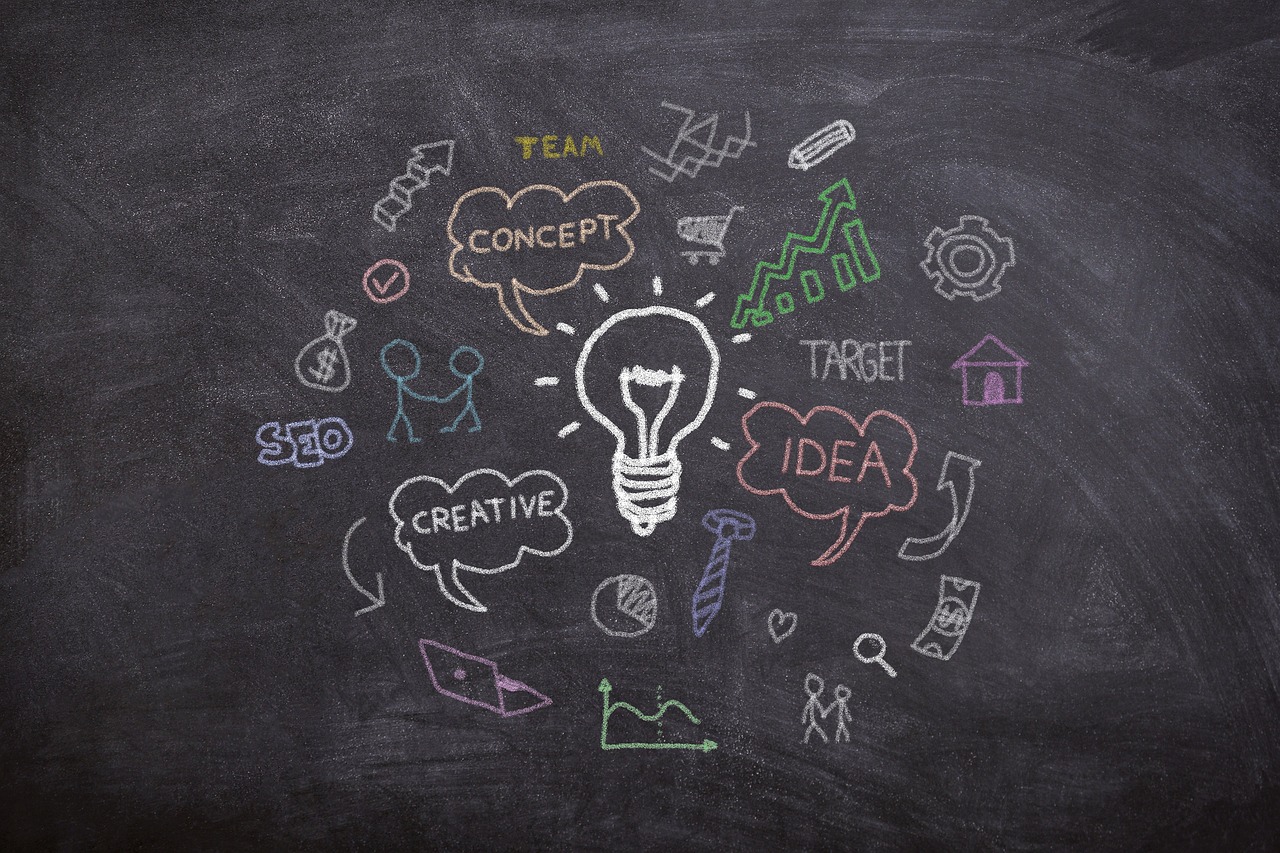
Ethical Considerations in AI Decision-Making
The rise of artificial intelligence (AI) in decision-making processes has opened up a realm of possibilities, but it also brings a host of ethical considerations that cannot be ignored. As AI systems become more integrated into our daily lives, questions about bias, transparency, and accountability arise, challenging us to think critically about how these technologies are developed and implemented.
One of the most pressing issues is bias in AI systems. Algorithms are only as good as the data they are trained on, and if that data reflects societal prejudices, the outcomes can be detrimental. For instance, if an AI system is trained on biased data regarding hiring practices, it may inadvertently favor one demographic over another, perpetuating inequality. This is particularly concerning in sectors like hiring, law enforcement, and lending, where biased decisions can have significant consequences for individuals and communities.
To tackle bias, organizations must adopt a proactive approach. Here are some strategies to consider:
- Diverse Data Sets: Ensure that the data used to train AI models is representative of various demographics to minimize bias.
- Regular Audits: Conduct audits of AI systems to identify and rectify biases in decision-making processes.
- Transparent Algorithms: Develop algorithms that are explainable, allowing users to understand how decisions are made.
Another critical ethical consideration is transparency. As AI systems become more complex, understanding how decisions are made can be challenging. This lack of transparency can lead to a distrust of AI, especially in high-stakes environments like healthcare or criminal justice. To combat this, organizations should strive to make their AI systems as transparent as possible, providing clear explanations of how decisions are reached and what data influences them.
Accountability is also a key concern in AI decision-making. When an AI system makes a mistake, who is responsible? Is it the developers, the organization using the AI, or the AI itself? Establishing clear lines of accountability is essential to ensure that individuals and organizations take responsibility for the outcomes of their AI systems. This could involve creating regulatory frameworks that outline the responsibilities of AI developers and users.
In summary, while AI offers immense potential to enhance decision-making processes, it is crucial to navigate the ethical landscape carefully. By addressing issues of bias, transparency, and accountability, we can harness the power of AI responsibly and equitably. The future of AI in decision-making will depend not only on technological advancements but also on our commitment to ethical principles that prioritize fairness and justice.
Q1: What is bias in AI decision-making?
A1: Bias in AI refers to the tendency of algorithms to produce unfair outcomes due to prejudiced data or flawed assumptions made during the design of the AI system.
Q2: How can organizations ensure transparency in AI systems?
A2: Organizations can ensure transparency by developing explainable AI systems that provide insights into how decisions are made and what data influences those decisions.
Q3: Who is accountable for AI decisions?
A3: Accountability for AI decisions typically falls on the developers and organizations that deploy the AI, but establishing clear regulatory frameworks can help clarify responsibilities.
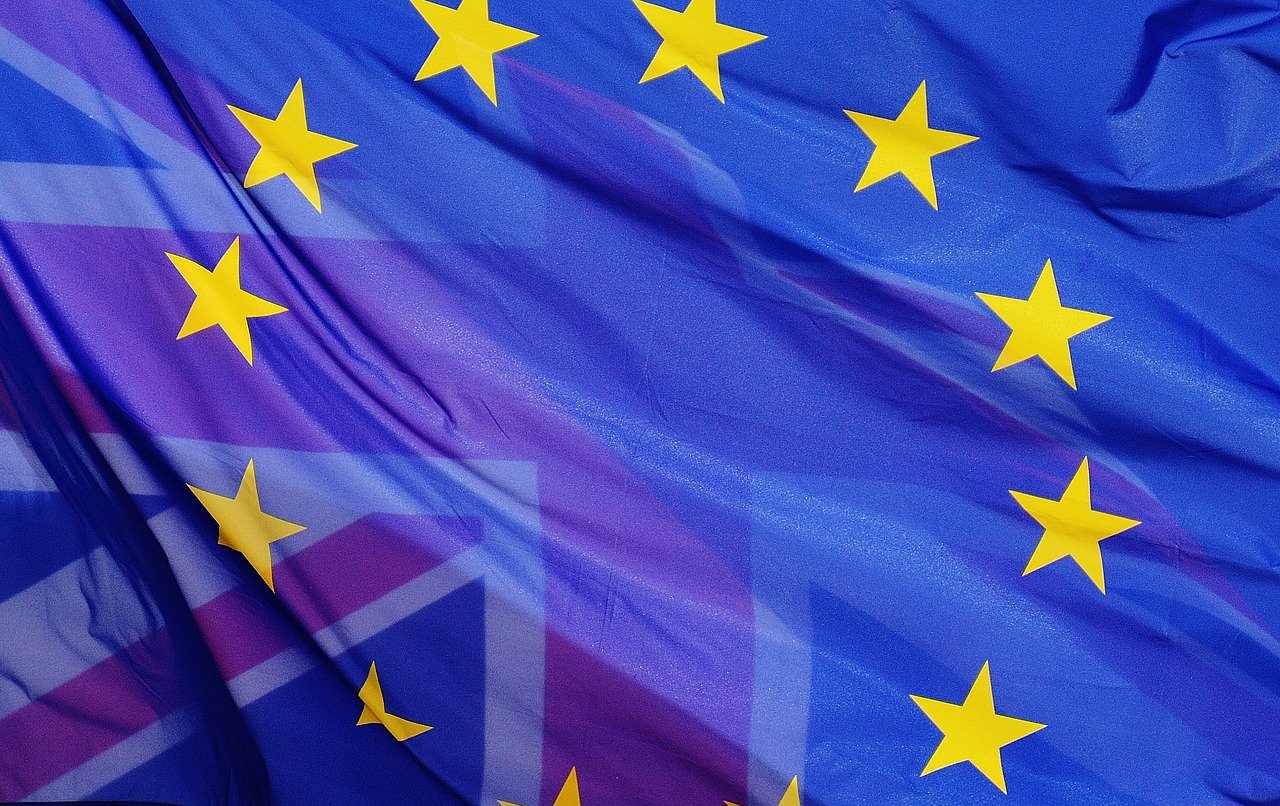
Addressing Bias in AI Systems
In today's rapidly advancing technological landscape, the issue of bias in artificial intelligence systems has emerged as a significant concern. Bias can seep into AI algorithms through various channels, often leading to unfair outcomes that affect individuals and communities. This is particularly troubling because AI systems are increasingly being used in critical areas such as hiring, law enforcement, and healthcare, where biased decisions can have serious ramifications. So, how do we tackle this pressing issue and ensure that AI serves all of humanity equitably?
First and foremost, it's essential to recognize that bias in AI is not merely a technical flaw; it often reflects societal biases that exist in the data used to train these systems. For instance, if an AI model is trained on historical data that contains gender or racial biases, it is likely to perpetuate those biases in its predictions. To combat this, organizations must prioritize diverse data collection methods. This means gathering data from a wide range of demographics and ensuring that minority groups are adequately represented. By doing so, we can create more balanced datasets that lead to fairer AI outcomes.
Another approach to addressing bias is through continuous monitoring and auditing of AI systems. Just like a car requires regular maintenance to run smoothly, AI algorithms need ongoing checks to ensure they are functioning as intended. This can involve setting up a dedicated team to regularly evaluate the performance of AI systems, looking specifically for signs of bias. In addition, employing explainable AI techniques can help demystify how decisions are made, allowing stakeholders to understand the rationale behind AI-driven outcomes. Transparency is key; when people can see how decisions are made, they are more likely to trust the system.
Furthermore, implementing algorithmic fairness techniques can help mitigate bias. These techniques can adjust the decision-making process to ensure that it does not favor one group over another. For example, some algorithms can be designed to equalize outcomes across different demographic groups, thereby reducing the impact of biased data. However, it’s crucial to strike a balance between fairness and accuracy; sometimes, a model that is perfectly fair might not perform as well in terms of accuracy. Thus, organizations must engage in thoughtful discussions about how to prioritize these competing goals.
Lastly, fostering a culture of diversity and inclusion within AI development teams is vital. When a team is composed of individuals from various backgrounds, they are more likely to identify potential biases that a homogenous group might overlook. Encouraging diverse perspectives can lead to more innovative solutions and more equitable AI systems. Education and training on bias and ethical AI practices should also be part of the team’s ongoing professional development.
Addressing bias in AI systems is not just a technical challenge; it is a societal imperative. By committing to diverse data collection, continuous monitoring, algorithmic fairness, and fostering diversity within teams, we can pave the way for AI systems that are not only intelligent but also just. It’s about creating a future where technology uplifts everyone rather than perpetuates existing inequalities.
- What is bias in AI? Bias in AI refers to systematic favoritism towards certain groups or outcomes due to flawed data or algorithms.
- How can organizations mitigate bias in AI systems? Organizations can mitigate bias by ensuring diverse data collection, continuous monitoring, and employing fairness techniques in their algorithms.
- Why is transparency important in AI? Transparency allows stakeholders to understand how decisions are made, fostering trust and accountability in AI systems.
- What role does diversity play in AI development? Diversity in AI development teams helps identify and address potential biases, leading to more equitable outcomes.
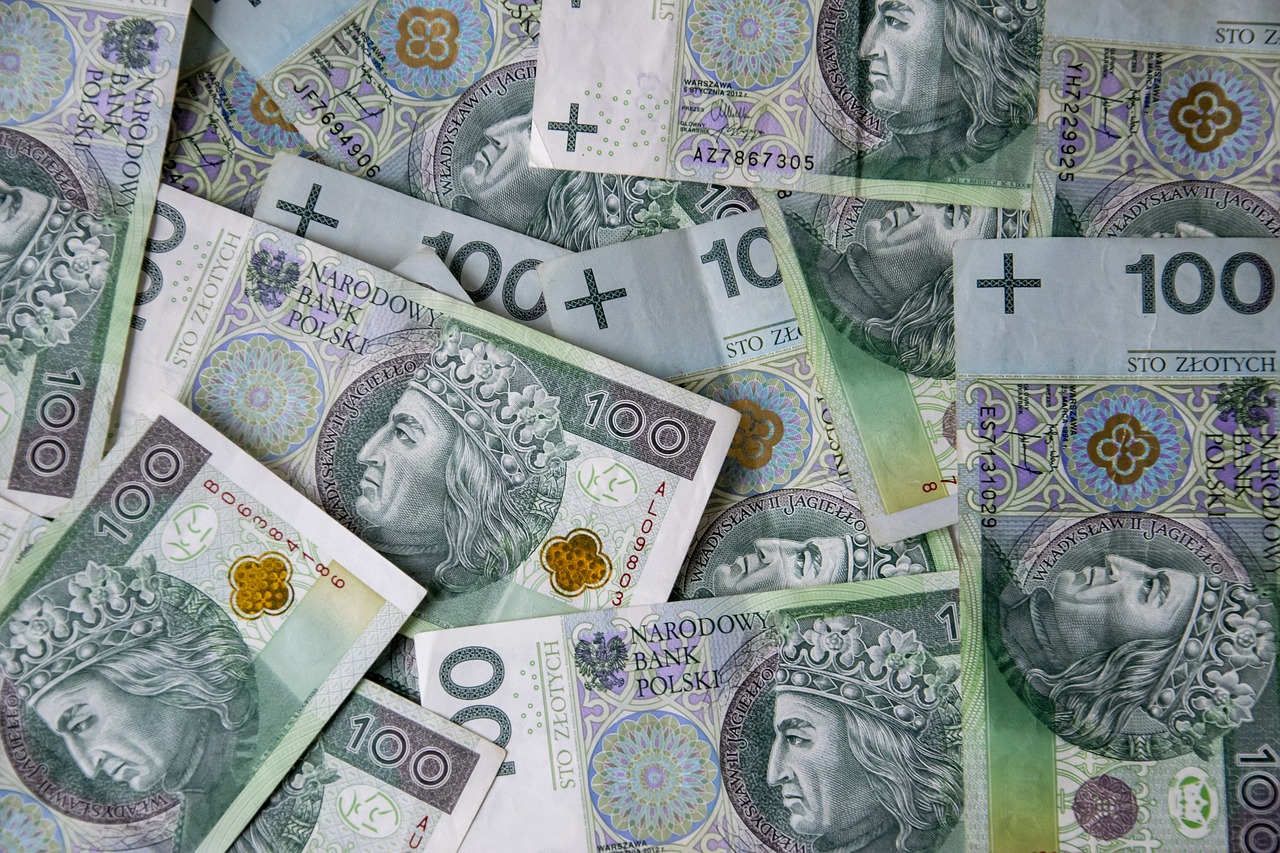
The Future of AI in Decision-Making
As we look towards the horizon, the role of artificial intelligence in decision-making is poised to evolve dramatically. Imagine a world where AI not only assists in making choices but also learns from past decisions, adapting and improving its recommendations over time. This future is not just a distant dream; it's on the verge of becoming our reality. With advancements in machine learning and data analytics, AI systems are becoming increasingly sophisticated, allowing for more nuanced and context-aware decision-making.
One of the most exciting prospects is the potential for greater human-AI collaboration. Picture this: a team of doctors working alongside AI algorithms that provide real-time insights and suggestions based on the latest medical research and patient data. This partnership can lead to more accurate diagnoses and tailored treatment plans, ultimately improving patient outcomes. The synergy between human intuition and AI's analytical prowess could redefine how we approach complex problems.
Moreover, as AI continues to integrate into various sectors, we can expect to see an increase in automated decision-making. This doesn't mean that humans will be entirely removed from the equation; rather, AI will handle routine decisions, freeing up human minds for more strategic and creative tasks. For instance, in the finance sector, AI can analyze market trends and execute trades based on predefined criteria, while human analysts can focus on developing innovative investment strategies.
However, this future isn't without its challenges. As AI systems become more autonomous, questions of accountability and transparency will loom large. Who is responsible when an AI makes a poor decision? How can we ensure that these systems operate fairly and without bias? Addressing these ethical concerns will be crucial as we navigate the evolving landscape of AI in decision-making.
To illustrate the potential trajectory of AI in decision-making, consider the following table that summarizes key areas where AI is expected to make significant impacts:
Sector | Impact of AI | Examples |
---|---|---|
Healthcare | Enhanced diagnostics and personalized treatment plans | AI-assisted imaging, predictive health analytics |
Finance | Automated trading and risk assessment | Algorithmic trading, fraud detection systems |
Retail | Personalized customer experiences and inventory management | Recommendation engines, demand forecasting |
Manufacturing | Predictive maintenance and supply chain optimization | Smart factories, AI-driven logistics |
In conclusion, the future of AI in decision-making promises to be both exciting and complex. As we harness the power of AI, we must also remain vigilant about the ethical implications and strive for systems that are not only efficient but also fair and transparent. Embracing this technology will require collaboration across disciplines, ensuring that as we move forward, we do so with a commitment to responsible innovation.
- Will AI completely replace human decision-making? No, AI is designed to assist and enhance human decision-making, not replace it.
- How can we ensure AI systems are unbiased? Regular audits, diverse training data, and transparency in algorithms are key to minimizing bias.
- What are the ethical concerns surrounding AI? Key concerns include accountability for decisions made by AI, transparency of algorithms, and potential biases in data.
- What industries will be most affected by AI in decision-making? Healthcare, finance, retail, and manufacturing are among the sectors expected to see significant changes.
Frequently Asked Questions
- What is the role of data in AI decision-making?
Data serves as the foundation for AI systems. It is collected, processed, and analyzed to influence the quality and effectiveness of AI-driven decisions across various industries. The better the data, the more accurate and reliable the decisions made by AI can be.
- How does AI enhance business strategy?
AI enhances business strategy by providing data-driven insights and predictive analytics that help organizations make informed decisions. By leveraging AI tools, businesses can gain a competitive edge, optimize operations, and respond more effectively to market changes.
- What are predictive analytics and how are they used?
Predictive analytics involves using historical data to forecast future trends. Businesses apply these techniques to anticipate customer behavior, manage risks, and make strategic decisions that can lead to improved outcomes and efficiency.
- Can you provide examples of successful AI implementations?
Sure! Many companies have successfully integrated predictive analytics into their decision-making processes. For instance, retailers use AI to analyze shopping patterns, while healthcare providers utilize it to improve patient outcomes by predicting disease progression.
- What challenges are associated with predictive analytics?
Despite its advantages, predictive analytics faces challenges such as data quality issues and model accuracy. Organizations must ensure they have high-quality data and continuously refine their models to maintain accuracy and reliability in predictions.
- How is AI transforming healthcare decision-making?
AI is revolutionizing healthcare by enhancing diagnostic accuracy and improving treatment planning. It aids clinicians in making better decisions, leading to improved patient outcomes and more efficient healthcare delivery.
- What are the ethical considerations in AI decision-making?
As AI systems become more prevalent, ethical concerns arise, particularly regarding bias, transparency, and accountability. It's crucial to address these issues to ensure that AI-driven decisions are fair and just.
- How can bias in AI systems be addressed?
Addressing bias in AI involves implementing strategies to identify and mitigate it in algorithms. This can include diversifying training data, regularly auditing AI systems, and ensuring transparency in how decisions are made.
- What does the future hold for AI in decision-making?
The future of AI in decision-making looks promising, with advancements in technology paving the way for greater human-AI collaboration. As AI continues to evolve, we can expect it to play an even more significant role in various sectors, enhancing the decision-making process.