The Role of AI in Personalizing User Experience
In today's digital age, where attention spans are shorter than ever, artificial intelligence (AI) is stepping up as a game changer. Imagine walking into a store where every product is tailored just for you, where every recommendation feels like it was handpicked by a friend. That's the magic of AI in personalizing user experiences across various platforms. It’s not just about making things look pretty; it’s about creating an environment where users feel understood and valued. By leveraging advanced algorithms and comprehensive data analysis, businesses are not only enhancing user engagement but also significantly improving customer satisfaction.
So, how does this work? Well, AI dives deep into the ocean of user data, analyzing preferences, habits, and interactions. This analysis enables businesses to tailor their offerings effectively, making each user’s journey unique and enjoyable. Just like a chef who knows their diners' tastes, AI serves up experiences that resonate on a personal level. This personalization is crucial because it transforms a generic experience into a memorable one, making customers more likely to return. But what exactly does this mean for users? Let’s explore further.
Understanding user behavior is like having a secret map to a treasure chest of insights. By analyzing how users interact with content, AI can uncover patterns that might not be immediately obvious. For instance, if a user frequently browses travel blogs, AI can infer that they have a keen interest in travel-related content. This understanding is vital for businesses that want to create personalized experiences that truly resonate with their audience.
Through data analytics, AI can categorize users into different segments based on their behaviors and preferences. This categorization allows businesses to craft targeted marketing strategies and offers. Think of it as a tailor who knows exactly what size and style suit you best. By aligning their offerings with user preferences, companies can enhance engagement and build lasting relationships with their customers.
When it comes to personalization, AI employs a variety of algorithms that work behind the scenes to deliver tailored experiences. Two of the most prominent methods are collaborative filtering and content-based filtering. Each of these approaches has its unique way of analyzing data patterns and predicting user preferences, ensuring that users receive recommendations that feel just right.
Collaborative filtering is like having a buddy who knows what you like based on the choices of others. It recommends products or content based on user interactions and preferences, allowing for a more tailored experience that resonates with individual users. For instance, if you and your friend both enjoy similar movies, collaborative filtering will suggest films that your friend liked, anticipating that you might enjoy them too.
This method enhances user satisfaction by providing personalized recommendations, increasing engagement, and fostering a sense of community among users with similar interests. Imagine logging into your favorite streaming service and being greeted with a list of shows that feel like they were curated just for you. That’s the beauty of collaborative filtering at work!
However, it's not all sunshine and rainbows. Collaborative filtering can face challenges like the cold start problem, where new users or items lack sufficient data for accurate recommendations. It’s a bit like trying to recommend a book to someone you've just met; without knowing their tastes, it’s tough to make a good suggestion.
On the other hand, content-based filtering focuses on recommending items similar to those a user has previously liked. If you’ve ever been on a shopping site and noticed that it suggests items similar to what you’ve previously purchased, you’ve experienced content-based filtering. This method ensures that users receive suggestions aligned with their established preferences, creating a seamless and enjoyable browsing experience.
AI-driven personalization significantly boosts customer engagement by delivering relevant content, improving interaction quality, and fostering loyalty through tailored experiences. When users see that a platform understands their needs and preferences, they are more likely to engage with the content and return for more.
One of the most exciting aspects of AI is its ability to provide real-time personalization. This means that as users interact with a website or app, AI can adapt the content instantly based on their behavior. For example, if a user shows interest in a specific product category, the platform can immediately highlight related products, creating a dynamic and engaging experience that meets users' immediate needs.
Personalized experiences created through AI can lead to higher customer retention rates. Users are more likely to return to platforms that consistently cater to their preferences. It’s akin to a favorite restaurant that remembers your go-to order; you’ll keep coming back because you feel valued and understood. In a world overflowing with options, personalization is the secret ingredient that keeps customers loyal.
- What is AI personalization? AI personalization refers to the use of artificial intelligence to tailor user experiences based on individual preferences and behaviors.
- How does collaborative filtering work? Collaborative filtering recommends items based on the preferences and behaviors of similar users.
- What are the challenges of AI personalization? Challenges include data privacy concerns and the cold start problem, where new users or items lack sufficient data for accurate recommendations.
- Can AI improve customer satisfaction? Yes, by providing personalized experiences, AI can significantly enhance customer satisfaction and engagement.
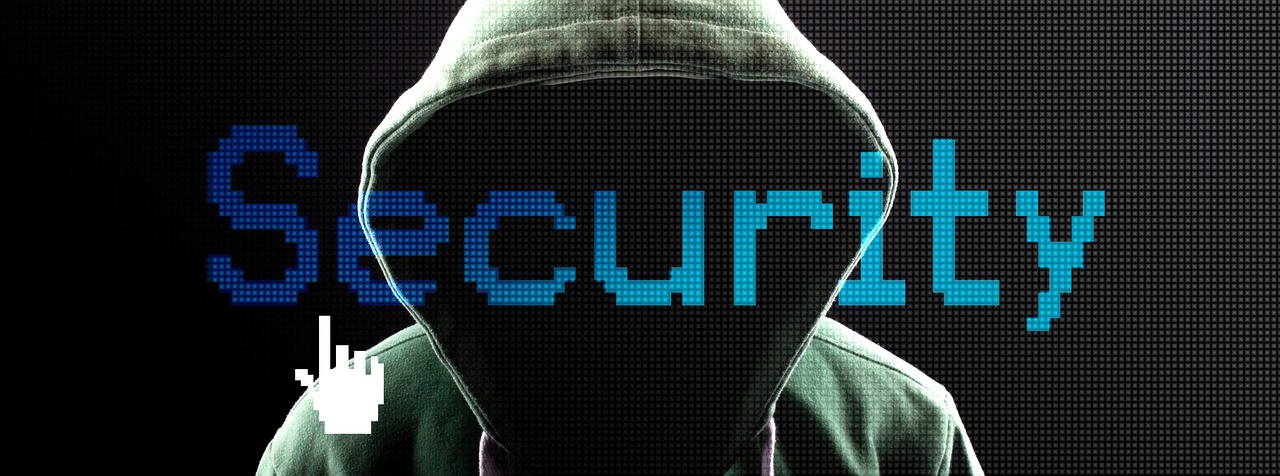
Understanding User Behavior
When it comes to personalizing user experiences, understanding user behavior is like having a treasure map leading you straight to the gold. It's not just about gathering data; it's about diving deep into the ocean of interactions, preferences, and habits that users exhibit across various platforms. With the help of artificial intelligence, companies can sift through mountains of data to uncover patterns that reveal what users truly want. Imagine a detective piecing together clues to solve a mystery; that's what AI does with user behavior data.
At the heart of this analysis lies the ability to track various metrics, such as:
- Click-through rates: Understanding which links attract users can help in crafting more engaging content.
- Time spent on site: The longer users stay, the more likely they are to find what they need.
- Purchase history: Knowing what users have bought in the past can inform future recommendations.
By employing advanced data analytics, AI can segment users based on their behaviors and preferences. This segmentation allows businesses to tailor their offerings more effectively. For instance, if a user frequently browses through sports gear, AI can highlight relevant promotions or new arrivals in that category. It’s like walking into a store where the sales associate knows your style and preferences, guiding you to exactly what you’re looking for.
Moreover, the insights gained from analyzing user behavior can inform marketing strategies, product development, and customer service approaches. Companies can create personalized campaigns that resonate with their target audience, leading to higher conversion rates and improved customer satisfaction. Think of it as a conversation where the brand is not just talking at the customer but engaging in a meaningful dialogue that addresses their needs and desires.
However, it’s essential to remember that while AI can analyze data, the human touch is irreplaceable. Understanding the emotional context behind user behavior is crucial. For example, a user might abandon their shopping cart not because they weren’t interested in the products, but due to unexpected life events or financial constraints. This is where empathy comes into play, allowing businesses to connect with users on a deeper level.
In conclusion, understanding user behavior through AI is a powerful tool for personalization. By leveraging data analytics, businesses can create tailored experiences that not only meet user expectations but also enhance overall satisfaction and loyalty. It’s a win-win situation where both users and brands benefit from a more personalized digital landscape.

AI Algorithms for Personalization
This article explores how artificial intelligence is transforming user experiences across various platforms by tailoring content, enhancing engagement, and improving customer satisfaction through advanced algorithms and data analysis.
Analyzing user behavior is crucial for personalizing experiences. AI utilizes data analytics to understand preferences, habits, and interactions, allowing businesses to tailor their offerings effectively.
When it comes to personalization, AI algorithms are the unsung heroes working behind the scenes. These algorithms are designed to sift through vast amounts of data, identifying patterns and trends that can help tailor user experiences. Think of them as digital detectives, piecing together clues from user interactions to create a custom-tailored experience that feels almost intuitive. Two of the most prominent methods in this realm are collaborative filtering and content-based filtering, each with its unique approach to understanding user preferences.
Collaborative filtering is like having a friend who knows your taste better than you do. By analyzing the behaviors of similar users, this algorithm recommends products or content based on what others with similar preferences have enjoyed. For instance, if you and a friend both loved a particular movie, collaborative filtering might suggest other films that your friend liked, assuming you'll enjoy them too. This method not only enhances user satisfaction but also creates a sense of community among users with shared interests.
The benefits of collaborative filtering are numerous:
- Personalized Recommendations: Users receive suggestions that are tailored to their unique tastes, making their experience more enjoyable.
- Increased Engagement: When users find content that resonates with them, they are more likely to spend time on the platform, leading to higher engagement rates.
- Community Building: By connecting users with similar interests, platforms can foster a sense of belonging and community.
However, collaborative filtering is not without its challenges. One significant hurdle is the cold start problem. This issue arises when new users or items lack sufficient data, making it difficult for the algorithm to provide accurate recommendations. Imagine walking into a new café where no one knows your favorite drink; it can be a bit daunting! To overcome this, platforms often implement strategies to gather initial data quickly, such as asking users to rate a few items during the sign-up process.
On the other side of the spectrum, we have content-based filtering. This method focuses on recommending items that are similar to those a user has previously liked. It’s akin to a personalized playlist that evolves based on your listening habits. If you’ve enjoyed a particular genre or artist, content-based filtering ensures that you receive suggestions that align with your established preferences. This approach can be particularly effective for users who have specific tastes, as it narrows down options to what they are likely to appreciate.
AI-driven personalization significantly boosts customer engagement by delivering relevant content, improving interaction quality, and fostering loyalty through tailored experiences.
Real-time personalization leverages AI to adapt content instantly based on user behavior, ensuring a dynamic and engaging experience that meets users' immediate needs.
Personalized experiences created through AI can lead to higher customer retention rates, as users are more likely to return to platforms that consistently cater to their preferences.
Q: How does AI improve user experience?
A: AI enhances user experience by analyzing data to provide personalized content and recommendations that align with individual preferences.
Q: What is the difference between collaborative filtering and content-based filtering?
A: Collaborative filtering recommends items based on user interactions and preferences, while content-based filtering suggests items similar to those a user has liked in the past.
Q: What challenges does collaborative filtering face?
A: One major challenge is the cold start problem, where new users or items lack sufficient data for accurate recommendations.

Collaborative Filtering
Collaborative filtering is a powerful method in the realm of artificial intelligence that offers personalized recommendations based on the collective preferences of users. Imagine walking into a bookstore where the staff knows your taste in literature so well that they can guide you straight to the latest bestsellers that match your interests. That’s the essence of collaborative filtering! It analyzes vast amounts of data from multiple users to identify patterns and similarities, allowing it to suggest products, services, or content that you might love.
This approach operates on the principle that if two users share similar tastes, they are likely to enjoy the same items. For instance, if User A and User B both loved a particular movie, and User A also liked another film that User B hasn’t seen yet, the system will recommend that film to User B. This creates a dynamic recommendation engine that evolves with user interactions, making it more effective over time.
Collaborative filtering can be divided into two main types: user-based and item-based. User-based collaborative filtering focuses on finding users with similar preferences and recommending items based on their likes. On the other hand, item-based collaborative filtering looks at the relationships between items themselves, suggesting similar items based on user interactions. Both methods leverage the power of community data to enhance the personalization experience.
However, while collaborative filtering has numerous advantages, it does come with some challenges. One significant issue is the cold start problem. This occurs when new users or items enter the system without enough data to generate accurate recommendations. Think of it like a new restaurant that has just opened; without reviews or customers, it’s hard to know what dishes will be popular. Similarly, without sufficient data, collaborative filtering struggles to provide relevant suggestions.
Despite these challenges, the benefits of collaborative filtering are substantial. It not only enhances user satisfaction by providing tailored recommendations but also increases engagement and fosters a sense of community among users with similar interests. By creating a more personalized experience, businesses can build stronger relationships with their customers, ultimately leading to higher retention rates.
As we continue to explore the impact of AI in personalizing user experiences, collaborative filtering stands out as a key player, transforming how we discover new content and products. With its ability to harness the collective wisdom of users, it opens up a world of possibilities for businesses looking to enhance their offerings and connect with their audience on a deeper level.
- What is collaborative filtering? Collaborative filtering is a method used in AI to recommend products or content based on the preferences and interactions of multiple users.
- How does collaborative filtering work? It analyzes data from users to identify patterns and similarities, enabling it to suggest items that users with similar tastes have liked.
- What are the types of collaborative filtering? The two main types are user-based and item-based collaborative filtering.
- What is the cold start problem? The cold start problem occurs when new users or items lack enough data for the system to generate accurate recommendations.
- What are the benefits of collaborative filtering? It enhances user satisfaction, increases engagement, and fosters community among users with shared interests.

Advantages of Collaborative Filtering
Collaborative filtering is like having a friend who knows exactly what you like and can recommend things tailored just for you. This method enhances user satisfaction significantly by providing personalized recommendations based on the preferences of similar users. Imagine walking into a bookstore where the staff already knows your taste in novels and can guide you to the latest releases that match your interests. That’s the magic of collaborative filtering!
One major advantage is the increased engagement it fosters. When users receive recommendations that resonate with their tastes, they are more likely to interact with the content or products. This heightened engagement not only keeps users coming back but also encourages them to explore more options, creating a rich, immersive experience. Think of it as being at a party where everyone shares your interests; it’s much more fun and engaging!
Moreover, collaborative filtering helps to build a sense of community among users. By analyzing the preferences of individuals with similar tastes, platforms can create a network effect where users feel connected to others who share their interests. This sense of belonging can lead to increased loyalty and participation in community discussions, reviews, and recommendations. Users often enjoy sharing their opinions and experiences, which can further enhance the platform's content and offerings.
Additionally, collaborative filtering can introduce users to content or products they may not have discovered on their own. This serendipitous discovery is akin to stumbling upon a hidden gem while browsing. Users are often surprised by how much they enjoy something they might not have chosen themselves, leading to a broader appreciation of the platform's offerings.
However, it’s important to note that the effectiveness of collaborative filtering relies heavily on the availability of sufficient user data. The more interactions and preferences that are recorded, the better the recommendations can be. Thus, platforms that successfully gather and analyze this data can create a powerful feedback loop, continuously improving the personalization process.
In summary, the advantages of collaborative filtering are multifaceted, contributing to enhanced user satisfaction, increased engagement, community building, and serendipitous discoveries. As users continue to enjoy tailored experiences, businesses can benefit from higher retention rates and a loyal customer base that feels understood and valued.
- What is collaborative filtering? Collaborative filtering is a method used to recommend products or content to users based on the preferences and interactions of similar users.
- How does collaborative filtering enhance user experience? It enhances user experience by providing personalized recommendations, which increases engagement and satisfaction.
- What are the challenges of collaborative filtering? One challenge is the cold start problem, where new users or items lack sufficient data for accurate recommendations.
- Can collaborative filtering work without a large user base? While it can work with a smaller user base, the effectiveness of collaborative filtering improves significantly with more data.

Challenges of Collaborative Filtering
While collaborative filtering is a powerful tool for enhancing user experiences, it does come with its set of challenges that can impact its effectiveness. One of the most significant hurdles is the cold start problem. This issue arises when new users or items are introduced into the system. Since collaborative filtering relies heavily on user interactions and historical data, new users may not have enough information for the algorithm to generate accurate recommendations. Imagine walking into a restaurant for the first time; the staff doesn’t know your preferences, making it hard for them to suggest a dish you’d love.
Another challenge is the data sparsity problem. In environments where user interactions are limited, the algorithm struggles to find meaningful patterns. For instance, if a platform has thousands of products but only a handful of users engaging with them, the recommendations can become less relevant. This situation can lead to a frustrating experience for users who might feel that the platform doesn't understand their needs.
Moreover, there’s the risk of popularity bias. Collaborative filtering tends to favor popular items, meaning that niche products might get overlooked. This bias can create a cycle where trending items receive more attention, while unique or lesser-known options fade into the background. As a result, users might miss out on discovering new favorites that align perfectly with their tastes.
Lastly, privacy concerns also come into play. As collaborative filtering requires extensive data collection about user behaviors and preferences, some users may feel uneasy about how their information is being used. This apprehension can lead to hesitance in sharing data, ultimately affecting the algorithm's ability to provide personalized recommendations. It's a delicate balance—how do you provide a tailored experience without crossing the line into invasive data collection?
In summary, while collaborative filtering offers exciting prospects for personalization, businesses must navigate these challenges carefully. By addressing the cold start problem, managing data sparsity, mitigating popularity bias, and respecting user privacy, companies can enhance the effectiveness of collaborative filtering and create more satisfying user experiences.
- What is collaborative filtering? Collaborative filtering is a method used to recommend items to users based on the preferences and behaviors of similar users.
- What are the main challenges of collaborative filtering? The main challenges include the cold start problem, data sparsity, popularity bias, and privacy concerns.
- How can businesses overcome the cold start problem? Businesses can use hybrid recommendation systems that combine collaborative filtering with content-based filtering to provide better recommendations for new users or items.
- Is user privacy a concern with collaborative filtering? Yes, user privacy is a significant concern as collaborative filtering relies on collecting user data to make recommendations.

Content-Based Filtering
Content-based filtering is a fascinating approach that focuses on recommending items that are similar to those a user has previously liked or interacted with. Imagine walking into a bookstore where the staff knows your taste so well that they can suggest titles that you would love, even before you ask! This is the essence of content-based filtering—leveraging the history of user preferences to provide suggestions that feel personalized and relevant. By analyzing the features of items that a user has shown interest in, AI can make educated guesses about what else they might enjoy, ensuring a seamless and engaging experience.
One of the key aspects of content-based filtering is its ability to adapt to individual preferences without relying on the behavior of other users. This is particularly beneficial for new users who may not have enough interaction data for collaborative filtering to work effectively. For instance, if a user has previously enjoyed action movies with strong female leads, the system can recommend other films that fit this description, even if no one else has watched them yet. This personalized approach not only enhances user satisfaction but also builds a more intimate connection between the user and the platform.
Moreover, content-based filtering can be enhanced through the use of advanced algorithms that analyze various attributes of items. These attributes can include genres, keywords, descriptions, and even user ratings. By creating a profile of the user’s preferences and matching it against a database of item features, the AI can generate a list of recommendations that are more likely to resonate with the user. In this way, the filtering process becomes a dynamic, evolving interaction that grows alongside the user’s tastes.
However, like any technology, content-based filtering has its limitations. For example, if a user has a very narrow set of interests, the recommendations may become repetitive over time. To combat this, platforms often combine content-based filtering with other methods, such as collaborative filtering, to offer a more rounded set of recommendations. This hybrid approach not only broadens the scope of suggestions but also keeps the user engaged by introducing them to new content that they might not have discovered otherwise.
In summary, content-based filtering is a powerful tool in the realm of personalized user experiences. By focusing on individual preferences and leveraging item attributes, AI can create a tailored experience that keeps users coming back for more. It’s like having a personal shopper who knows your style inside and out, always ready with the perfect recommendations that make you feel understood and valued.
Frequently Asked Questions
- What is content-based filtering?
Content-based filtering is a recommendation system that suggests items similar to those a user has previously liked, based on the attributes of the items.
- How does content-based filtering work?
It analyzes the features of previously liked items and compares them to a database of other items to generate personalized recommendations.
- What are the advantages of content-based filtering?
It provides personalized recommendations, is effective for new users, and helps maintain user engagement by adapting to individual preferences.
- Are there any challenges with content-based filtering?
Yes, it can lead to repetitive recommendations if a user has narrow interests, which is why many platforms use a hybrid approach.

Enhancing Customer Engagement
In today's fast-paced digital landscape, customer engagement is paramount. With the rise of artificial intelligence (AI), businesses are finding innovative ways to connect with their audiences on a deeper level. Imagine walking into a store where every product seems to speak directly to your needs and desires. That's what AI-driven personalization can achieve in the online world. By analyzing user data, AI can deliver content that resonates with individual preferences, ensuring that every interaction feels meaningful and tailored.
One of the most exciting aspects of AI is its ability to provide real-time personalization. This means that as users navigate a platform, AI can adjust the content they see based on their immediate actions and interests. For instance, if a user spends more time looking at fitness gear, the platform can instantly showcase related products, articles, or even workout videos. This dynamic interaction not only keeps users engaged but also makes them feel understood and valued. It's like having a personal shopping assistant who knows your style and preferences inside out!
Moreover, AI enhances customer engagement by improving the quality of interactions. By utilizing advanced algorithms, businesses can analyze past behavior to predict future needs. For example, if a user frequently browses travel destinations, the system might suggest tailored travel packages or exclusive deals, creating a sense of anticipation and excitement. This proactive approach not only increases the likelihood of conversion but also fosters loyalty, as customers appreciate being recognized and catered to.
To illustrate the impact of AI on customer engagement, consider the following table:
Engagement Method | AI Contribution | Customer Benefit |
---|---|---|
Real-Time Recommendations | Instantly analyzes user behavior | Relevant content delivery |
Personalized Emails | Tailors messages based on preferences | Increased open rates and engagement |
Chatbots | Provides immediate customer support | Enhanced user satisfaction |
As we dive deeper into the world of AI, it's crucial to recognize its profound impact on customer retention. When users feel that their preferences are consistently catered to, they're more likely to return to a platform. It's akin to a favorite restaurant that remembers your usual order; the sense of familiarity and care builds a strong bond. This is why businesses leveraging AI for personalization often see a significant uptick in customer loyalty and retention rates.
In conclusion, enhancing customer engagement through AI is not just about technology; it's about creating a human connection in a digital space. By delivering relevant content, adapting in real-time, and improving interaction quality, businesses can foster a loyal customer base that feels valued and understood. As we continue to embrace the capabilities of AI, the future of customer engagement looks not only promising but also incredibly exciting.
- What is AI-driven personalization? AI-driven personalization refers to the use of artificial intelligence to tailor content and experiences to individual users based on their preferences and behaviors.
- How does real-time personalization work? Real-time personalization analyzes user actions as they happen, allowing businesses to adjust content and recommendations instantly to enhance the user experience.
- Why is customer engagement important? Customer engagement is crucial because it fosters loyalty, increases retention rates, and ultimately drives sales and revenue for businesses.
- What are some common AI algorithms used for personalization? Common algorithms include collaborative filtering, content-based filtering, and machine learning models that analyze user data patterns.
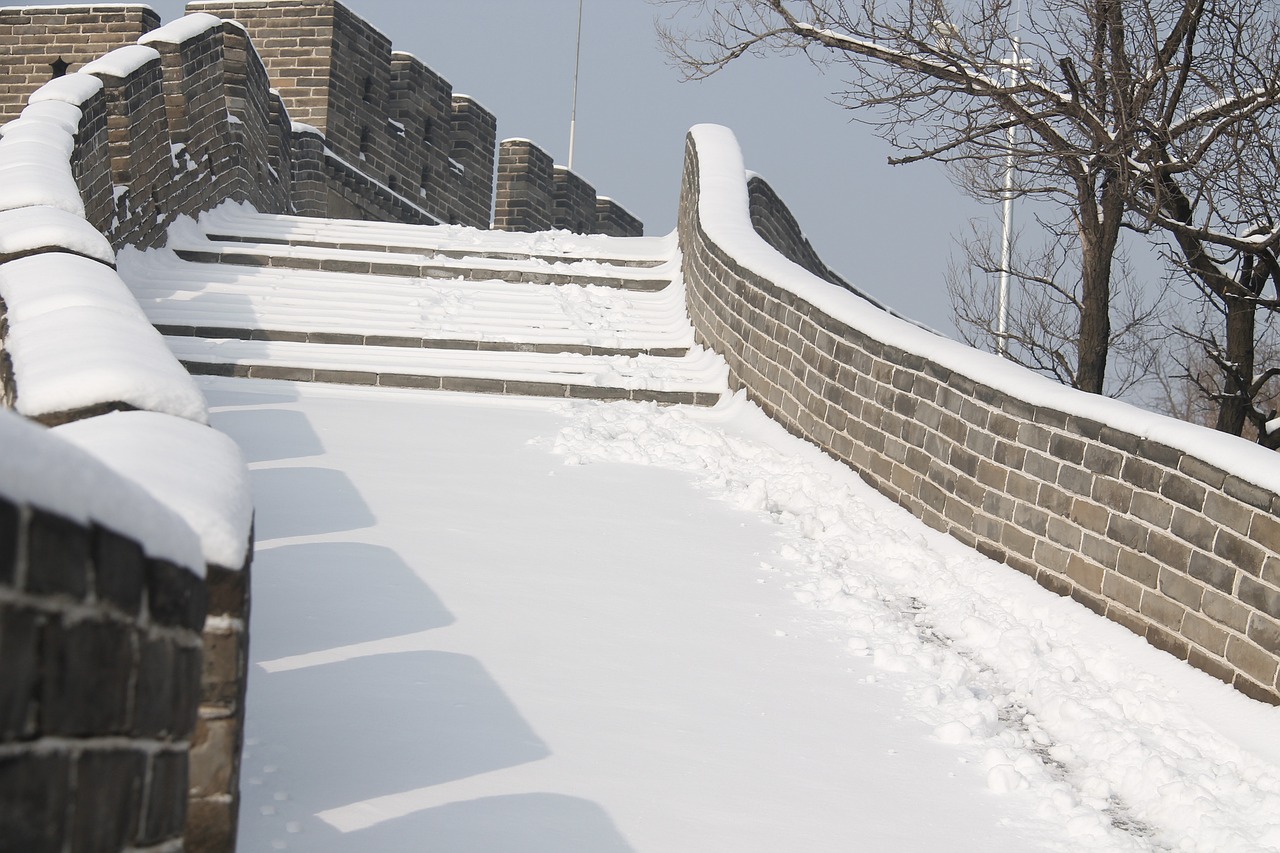
Real-Time Personalization
In today’s fast-paced digital world, has become a game-changer for businesses aiming to capture and retain customer attention. Imagine walking into a store where the staff immediately recognizes your preferences and suggests products tailored just for you. That’s the magic of real-time personalization, but in the online realm. By leveraging the power of artificial intelligence, companies can analyze user behavior as it happens, allowing them to modify content, offers, and recommendations instantly. This not only creates a more engaging experience for users but also significantly increases the likelihood of conversions.
One of the key aspects of real-time personalization is its ability to respond to user actions almost instantaneously. For instance, if a user spends a bit more time on a specific category of products on an e-commerce site, AI algorithms can quickly recognize this behavior and adjust the displayed content accordingly. This could mean showcasing similar items, offering discounts, or even sending personalized notifications. The result? A seamless shopping experience that feels intuitive and customized.
To illustrate how real-time personalization works, consider the following table that highlights various triggers and corresponding personalized actions:
User Trigger | Personalized Action |
---|---|
Browsing a specific product category | Display similar products and relevant reviews |
Abandoning a shopping cart | Send a reminder email with a discount offer |
Returning to the site after a break | Showcase new arrivals or items based on past purchases |
This level of personalization not only enhances user satisfaction but also builds a sense of loyalty. When users feel that their needs are understood and prioritized, they are more likely to return to the platform. Moreover, real-time personalization fosters a dynamic interaction where users feel they are part of a conversation rather than just passive consumers.
However, achieving effective real-time personalization requires a robust infrastructure that can handle vast amounts of data swiftly. Companies need to invest in advanced data analytics tools and ensure that their systems are capable of processing user interactions in real-time. This can pose challenges, especially for smaller businesses, but the potential rewards—such as increased customer engagement and higher conversion rates—make it a worthwhile endeavor.
In conclusion, real-time personalization is not just a trend; it’s a necessity for businesses that want to thrive in a competitive landscape. By understanding user behavior and leveraging AI technologies, companies can create an engaging, tailored experience that resonates with their audience, ultimately driving growth and success.
- What is real-time personalization? It refers to the ability to adapt content and recommendations instantly based on user behavior and preferences.
- How does AI contribute to real-time personalization? AI analyzes user data and interactions to provide tailored experiences that meet immediate needs.
- What are the benefits of real-time personalization? It enhances user engagement, increases conversion rates, and fosters customer loyalty.
- What challenges are associated with real-time personalization? The need for advanced data processing capabilities and potential privacy concerns can pose challenges.

Impact on Customer Retention
When we talk about customer retention, we're diving into the heart of what keeps businesses alive and thriving. In today's fast-paced digital world, where competition is just a click away, maintaining a loyal customer base is more crucial than ever. This is where the magic of artificial intelligence comes into play. By leveraging AI-driven personalization, businesses can create experiences that resonate deeply with users, ultimately leading to higher retention rates.
Imagine walking into your favorite coffee shop. The barista already knows your name and your usual order. This personal touch makes you feel valued and appreciated, right? Now, think of how that same concept applies to online platforms. When a user feels understood and catered to, they're more likely to return. AI achieves this by analyzing user behavior and preferences, allowing businesses to tailor their offerings in real-time. This leads to a more engaging experience that fosters loyalty.
Moreover, personalized recommendations can significantly enhance user satisfaction. For instance, when a streaming service suggests a movie based on your viewing history, it feels like they know you personally. This kind of tailored experience not only keeps users coming back but also encourages them to explore more content, increasing their overall engagement with the platform.
To illustrate the impact of AI on customer retention, consider the following table:
Aspect | Traditional Approach | AI-Driven Approach |
---|---|---|
Understanding Preferences | Generic surveys | Real-time data analysis |
Recommendations | Manual curation | Personalized suggestions based on behavior |
User Engagement | Standard promotions | Dynamic content tailored to user interests |
Retention Strategy | One-size-fits-all | Customized loyalty programs |
As shown in the table, the shift from traditional methods to AI-driven strategies can revolutionize how businesses approach customer retention. By utilizing advanced algorithms, companies can create a more personalized experience that not only meets but anticipates user needs. This proactive approach fosters a deeper connection between the brand and its customers.
Furthermore, AI can also help identify at-risk customers. By analyzing patterns in user behavior, businesses can pinpoint individuals who may be disengaging. This insight allows for timely interventions—like targeted offers or personalized communication—that can reignite interest and encourage users to stay loyal to the brand.
In conclusion, the impact of AI on customer retention is profound. By crafting personalized experiences that resonate with users, businesses can build lasting relationships that go beyond mere transactions. In a world where attention is fleeting, AI provides the tools needed to keep customers engaged and coming back for more.
- How does AI improve customer retention? AI enhances customer retention by personalizing user experiences, predicting preferences, and providing timely recommendations based on user behavior.
- What are the challenges of implementing AI for retention? Challenges can include data privacy concerns, the need for high-quality data, and the complexity of integrating AI systems into existing workflows.
- Can small businesses benefit from AI-driven personalization? Absolutely! Small businesses can leverage AI tools to understand their customers better and create personalized experiences that can compete with larger companies.
Frequently Asked Questions
- What is the role of AI in personalizing user experiences?
AI plays a crucial role in personalizing user experiences by analyzing vast amounts of data to understand user preferences and behaviors. This enables businesses to tailor their content, recommendations, and interactions, ultimately enhancing customer satisfaction and engagement.
- How does AI analyze user behavior?
AI utilizes advanced data analytics techniques to track and analyze user interactions across various platforms. By examining patterns in user behavior, such as clicks, purchases, and browsing history, AI can identify preferences and habits, allowing for more personalized offerings.
- What are collaborative filtering and content-based filtering?
Collaborative filtering is an AI technique that recommends products or content based on the preferences and interactions of similar users. In contrast, content-based filtering suggests items that are similar to those a user has previously liked, focusing on individual user preferences rather than group behavior.
- What are the advantages of collaborative filtering?
Collaborative filtering enhances user satisfaction by providing tailored recommendations, increasing engagement, and creating a sense of community among users with similar interests. This method allows for a more personalized experience that resonates with individual users, leading to improved customer loyalty.
- What challenges does collaborative filtering face?
One of the main challenges of collaborative filtering is the cold start problem, which occurs when new users or items lack sufficient data for accurate recommendations. This can make it difficult to provide personalized suggestions until enough data is collected.
- How does real-time personalization work?
Real-time personalization leverages AI to adapt content instantly based on user behavior. This means that as users interact with a platform, AI can modify the content they see in real-time, ensuring a dynamic and engaging experience that meets their immediate needs.
- What impact does AI-driven personalization have on customer retention?
AI-driven personalization can significantly boost customer retention rates. When users receive tailored experiences that cater to their preferences, they are more likely to return to platforms that consistently meet their needs, fostering loyalty and long-term relationships.