How AI is Enhancing Environmental Monitoring
In today's fast-paced world, the need for effective environmental monitoring has never been more pressing. With the rise of artificial intelligence (AI), we are witnessing a **revolution** in how we understand and manage our natural resources. AI is not just a buzzword; it's a powerful tool that is reshaping environmental science. From analyzing vast datasets to predicting future ecological changes, AI is enhancing our ability to monitor and respond to environmental challenges like never before.
Imagine having the capability to predict pollution levels in real-time or to monitor the health of our oceans and forests with just a few clicks. This is no longer a distant dream; it is a reality made possible through AI technologies. By harnessing the power of machine learning and data analytics, scientists and researchers can now gain insights that were previously unattainable. This transformation is crucial as we face pressing issues such as climate change, biodiversity loss, and pollution.
As we dive deeper into the applications of AI in environmental monitoring, it's essential to recognize its **multifaceted benefits**. For instance, AI can process and analyze data from various sources, including satellites, sensors, and social media, providing a comprehensive view of environmental conditions. This capability not only enhances our understanding of current situations but also aids in forecasting future trends. Moreover, AI's ability to identify patterns and anomalies in data allows for quicker responses to environmental crises, which can be the difference between a minor issue and a full-blown disaster.
Furthermore, the integration of AI in environmental monitoring is fostering **collaboration** among various stakeholders, including governments, NGOs, and private sectors. This collective effort is vital in creating policies that are informed by accurate data and predictive models. As we continue to explore how AI is transforming environmental monitoring, we also need to consider its implications for sustainability and conservation efforts on a global scale. The future is bright, and with AI at the helm, we are better equipped to tackle the environmental challenges that lie ahead.
- What is AI in environmental monitoring?
AI refers to the use of advanced algorithms and machine learning techniques to analyze and interpret environmental data, enhancing our ability to monitor and manage natural resources effectively. - How does AI improve air quality monitoring?
AI algorithms analyze real-time air quality data, predict pollution levels, and identify sources of contaminants, helping to create healthier urban environments. - Can AI help in wildlife conservation?
Yes, AI technologies are used to monitor animal populations, track movements, and identify threats, thereby supporting efforts to protect endangered species and their habitats. - What are the challenges of using AI in environmental monitoring?
Some challenges include data privacy concerns, potential algorithm bias, and the need for interdisciplinary collaboration to ensure effective implementation. - What does the future hold for AI in environmental sustainability?
The future looks promising, with AI expected to drive innovations in monitoring practices and support global efforts to combat climate change and biodiversity loss.
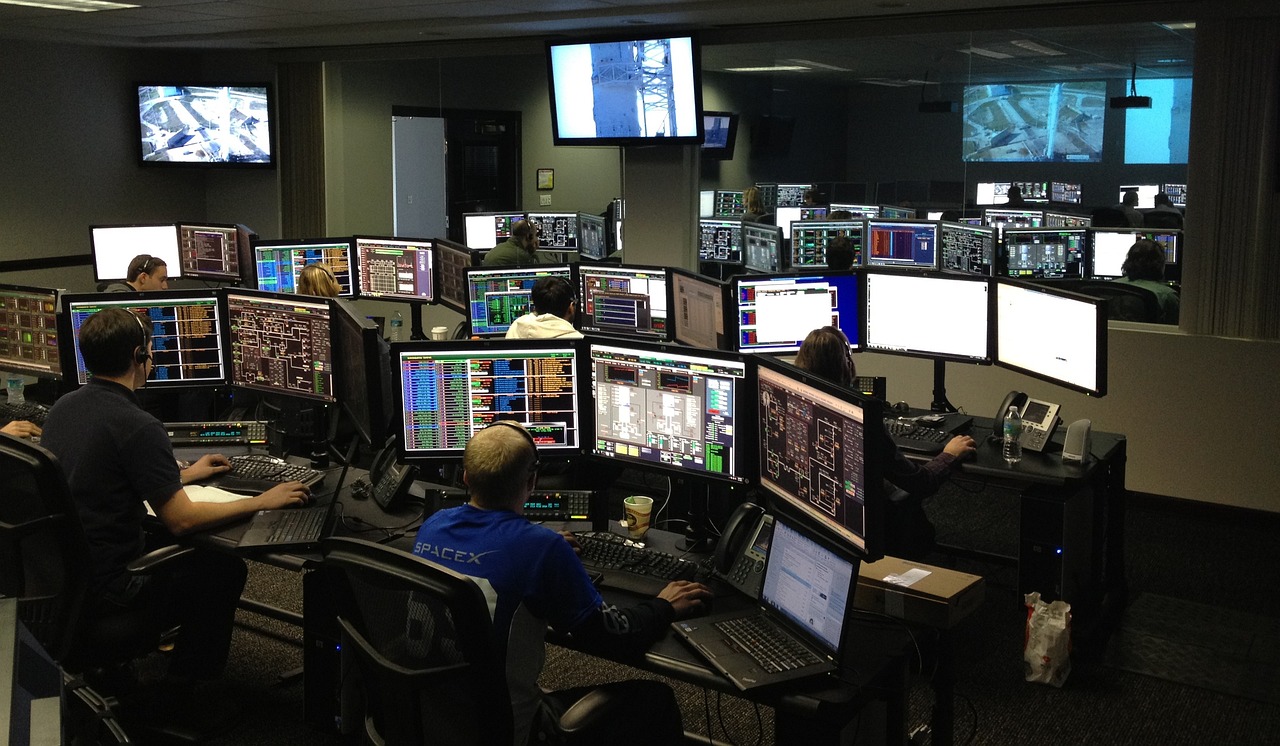
Understanding AI in Environmental Science
Artificial Intelligence (AI) is not just a buzzword; it's a game-changer in the realm of environmental science. Imagine having a super-smart assistant that can sift through mountains of data in seconds, spotting trends and anomalies that would take humans weeks or even months to uncover. That's the power of AI! By leveraging advanced algorithms and machine learning, AI is enabling researchers and environmentalists to gain a deeper understanding of our planet's complex ecosystems.
One of the most fascinating aspects of AI in environmental science is its ability to analyze vast datasets collected from various sources, such as satellites, sensors, and ground-based observations. This data can include anything from temperature readings and humidity levels to pollutant concentrations and biodiversity indices. By applying AI, scientists can not only process this information more efficiently but can also uncover hidden patterns that inform our understanding of environmental changes.
For instance, AI can help predict how ecosystems respond to different stressors, such as climate change or urban development. By creating predictive models, researchers can simulate various scenarios and assess potential outcomes. This capability is crucial for developing effective conservation strategies and for making informed policy decisions. Imagine being able to foresee the impacts of deforestation or pollution before they happen—AI makes that possible!
Moreover, AI enhances real-time monitoring of environmental conditions. With the integration of Internet of Things (IoT) devices, data can be collected continuously, providing a dynamic view of our environment. This real-time data stream allows for immediate responses to environmental threats, such as toxic spills or sudden changes in air quality. The ability to act swiftly can mean the difference between a minor incident and a full-blown environmental disaster.
However, it's important to note that the effectiveness of AI in environmental science does not solely hinge on technology. It requires collaboration across various disciplines, including ecology, data science, and public policy. By working together, experts can ensure that AI tools are used to their fullest potential, driving meaningful change in how we monitor and protect our planet.
In summary, AI is revolutionizing environmental science by:
- Enhancing data analysis capabilities
- Providing predictive modeling for better decision-making
- Facilitating real-time monitoring
- Encouraging interdisciplinary collaboration
This transformative technology is paving the way for a more sustainable future, where we can not only understand our environment better but also take proactive steps to safeguard it for generations to come.
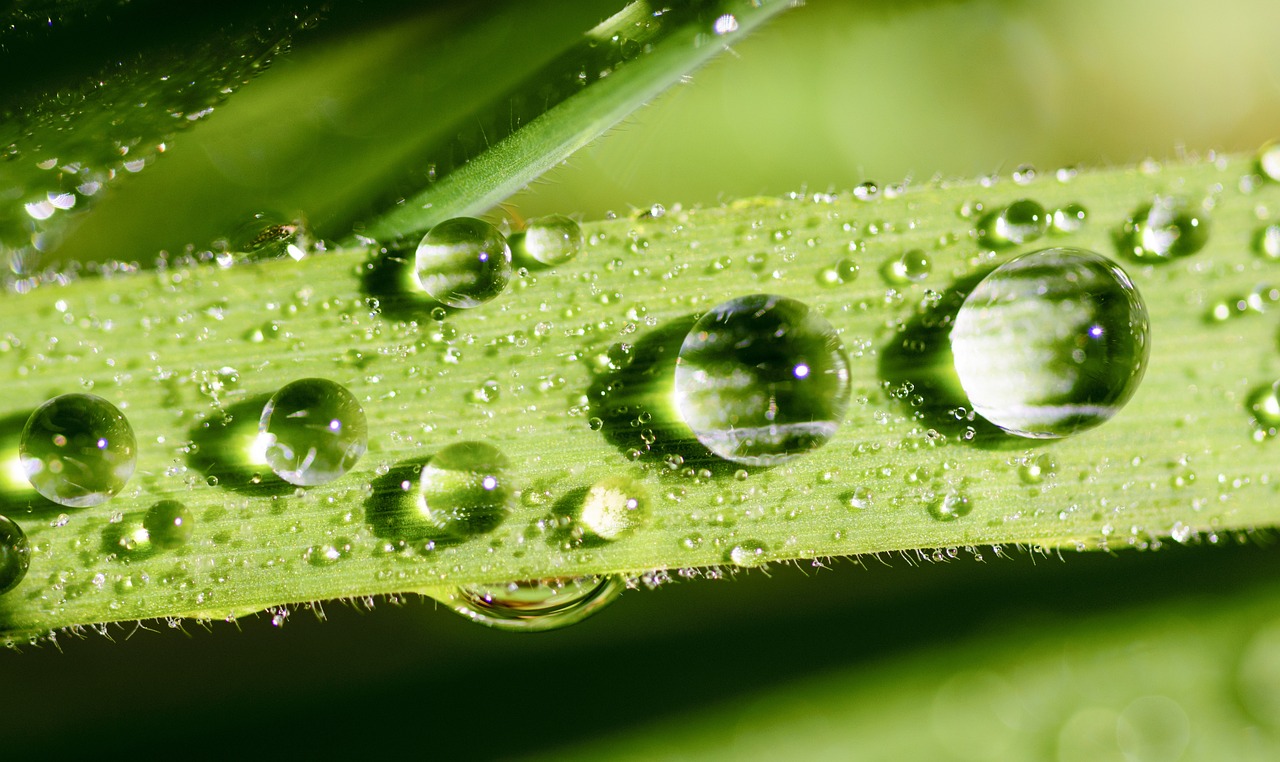
Applications of AI in Monitoring Air Quality
The quality of the air we breathe is crucial for our health and well-being, yet it remains one of the most challenging environmental issues we face today. With urbanization on the rise and industrial emissions contributing to pollution, understanding air quality has never been more critical. This is where artificial intelligence (AI) steps in, revolutionizing how we monitor and manage air quality. By leveraging advanced algorithms and data analytics, AI provides innovative solutions that enhance our ability to predict pollution levels and identify sources of contaminants.
One of the most exciting applications of AI in air quality monitoring is its ability to analyze large datasets from various sources, including satellite imagery, ground sensors, and social media feeds. These datasets can be overwhelming, but AI algorithms excel at sifting through this information to detect patterns and trends. For example, AI can predict pollution spikes based on historical data, weather conditions, and traffic patterns, allowing city planners and policymakers to take proactive measures. Imagine being able to forecast when air quality will deteriorate, enabling communities to prepare and respond effectively!
Moreover, AI-powered systems can identify specific sources of pollution. By using machine learning techniques, these systems can analyze emissions data from factories, traffic patterns, and even agricultural activities. This means that instead of just knowing that pollution exists, we can pinpoint where it’s coming from and take targeted action. For instance, if a factory is found to be a significant contributor to local air pollution, regulators can implement stricter controls or encourage cleaner technologies. This level of precision is a game-changer in the fight for cleaner air.
Another fascinating aspect of AI in air quality monitoring is its integration with Internet of Things (IoT) devices. Smart sensors placed throughout urban areas can continuously collect air quality data and transmit it in real-time. AI algorithms can analyze this data on-the-fly, providing immediate insights into air quality fluctuations. This real-time monitoring allows communities to receive alerts about unhealthy air conditions, enabling individuals to make informed choices about outdoor activities. Imagine receiving a notification on your phone telling you that air quality is poor in your area, prompting you to stay indoors or wear a mask!
Furthermore, AI is enhancing public engagement and awareness regarding air quality issues. With user-friendly interfaces and visualizations, AI-powered applications can present complex air quality data in a digestible format for the general public. This transparency fosters community involvement and encourages citizens to advocate for healthier environments. When people are informed about air quality conditions, they are more likely to support policies aimed at reducing pollution.
However, it’s important to note that the implementation of AI in air quality monitoring is not without its challenges. Data privacy concerns, algorithm bias, and the need for accurate, high-quality data are all hurdles that need to be addressed. While AI offers incredible potential, it must be used responsibly to ensure that it serves the public good without compromising individual rights.
In conclusion, the applications of AI in monitoring air quality are vast and varied. From predictive analytics to real-time data processing, AI is transforming how we understand and manage air quality. As we continue to harness the power of technology, we can look forward to cleaner air and healthier communities. The future of air quality monitoring is bright, and with AI leading the charge, we can make significant strides towards a more sustainable world.
- How does AI improve air quality monitoring? AI enhances air quality monitoring by analyzing large datasets to predict pollution levels and identify sources of contaminants.
- What role do IoT devices play in air quality monitoring? IoT devices collect real-time air quality data, which AI algorithms analyze to provide immediate insights and alerts.
- Are there any challenges associated with using AI for air quality monitoring? Yes, challenges include data privacy concerns, algorithm bias, and the need for high-quality data.
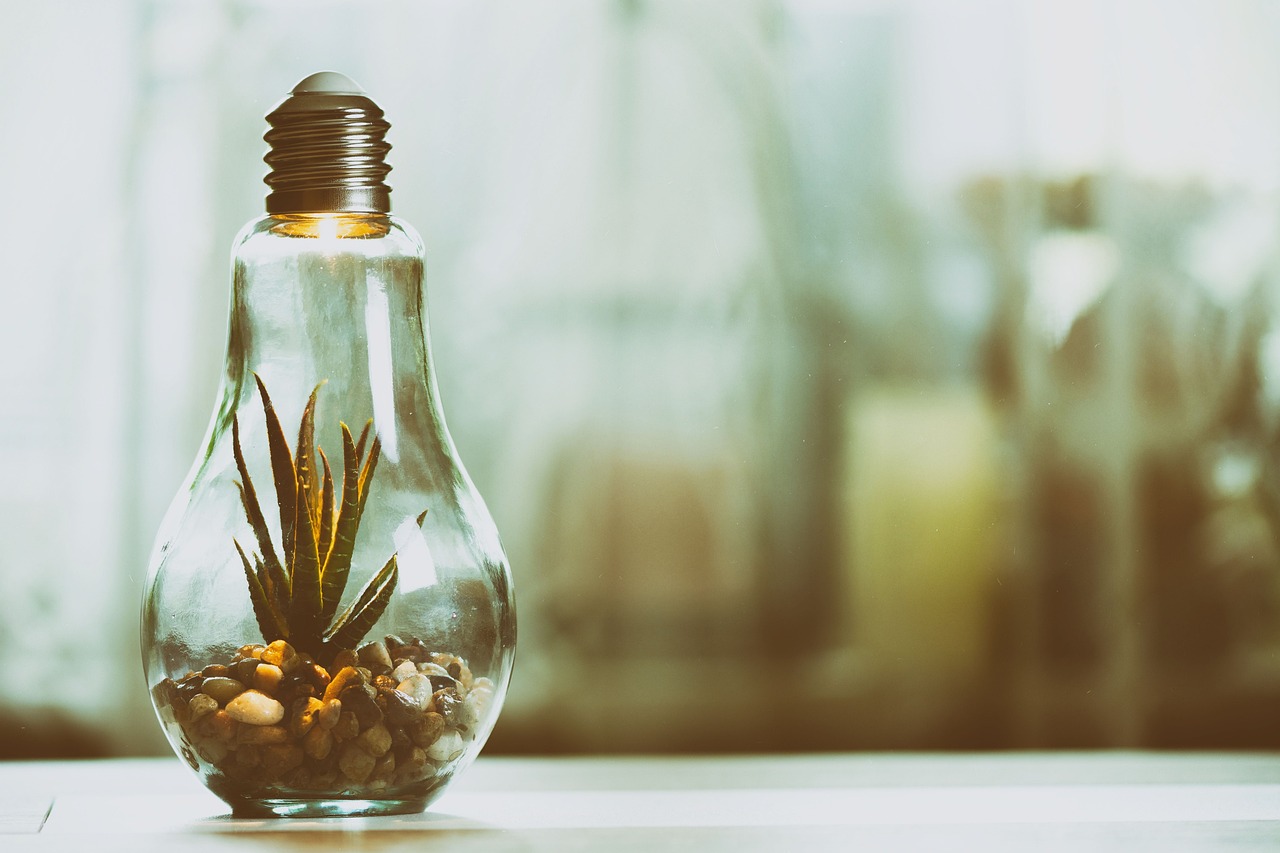
AI for Water Quality Assessment
Artificial Intelligence (AI) is making significant strides in the realm of water quality assessment, revolutionizing how we monitor and manage our precious water resources. Traditionally, assessing water quality required extensive fieldwork, manual sampling, and time-consuming laboratory analyses. However, with the advent of AI technologies, this process has become more efficient, accurate, and timely. Imagine a world where real-time data on water quality is available at our fingertips, enabling us to make informed decisions that protect both human health and aquatic ecosystems. Sounds like science fiction? Well, it's happening right now!
One of the most exciting applications of AI in water quality assessment is the use of machine learning algorithms. These algorithms can analyze vast amounts of data collected from various sources, such as sensors deployed in rivers, lakes, and reservoirs. By processing this data, AI can detect patterns and anomalies that might indicate the presence of pollutants, harmful bacteria, or other water quality issues. For instance, when a sudden spike in turbidity levels is detected, AI can alert authorities to potential contamination, allowing for rapid response measures to be implemented.
Moreover, AI can integrate data from multiple sources, including satellite imagery and weather forecasts, to provide a comprehensive picture of water quality across different regions. This capability is vital for managing water resources in an era of climate change, where extreme weather events can drastically affect water quality. By leveraging predictive analytics, AI can forecast potential water quality issues before they escalate, enabling proactive measures to safeguard our water bodies.
In addition to monitoring, AI also plays a crucial role in data interpretation. The algorithms can sift through complex datasets to identify the root causes of water quality degradation. For example, if a particular area is experiencing increased levels of nitrates, AI can analyze land use patterns, agricultural practices, and rainfall data to pinpoint the sources of pollution. This insight is invaluable for policymakers and environmental managers looking to implement effective mitigation strategies.
Despite these advancements, there are still challenges to overcome in the integration of AI into water quality assessment. Data quality and availability can vary significantly, which can affect the accuracy of AI models. Additionally, there is a need for interdisciplinary collaboration between environmental scientists, data analysts, and policymakers to ensure that AI solutions are tailored to specific regional needs and regulatory frameworks.
To illustrate the impact of AI on water quality assessment, consider the following table that highlights some successful implementations:
Project | Location | AI Application | Outcome |
---|---|---|---|
Smart Water Management | California, USA | Real-time monitoring using IoT sensors and machine learning | Reduced water waste by 30% |
Water Quality Prediction | Lake Victoria, Africa | Predictive analytics for algal blooms | Improved response time to water quality threats |
Pollution Source Tracking | Thames River, UK | Data integration from multiple sources | Identified key pollution sources for targeted action |
In conclusion, AI is not just a buzzword; it is a powerful tool that is reshaping how we assess and manage water quality. By harnessing the potential of AI, we can ensure cleaner, safer water for all, while also protecting our vital aquatic ecosystems. The future of water quality assessment is bright, and with continued innovation and collaboration, we can tackle the challenges ahead with confidence.
- How does AI improve water quality monitoring? AI enhances monitoring by analyzing large datasets in real-time, detecting anomalies, and predicting potential contamination events.
- What types of data are used in AI water quality assessments? Data sources include sensor readings, satellite imagery, weather data, and historical water quality records.
- Are there any limitations to using AI for water quality assessment? Yes, challenges include data quality, algorithm bias, and the need for collaboration among various stakeholders.
- Can AI help in responding to water quality crises? Absolutely! AI can provide early warnings and predictive insights that enable quicker response to water quality issues.
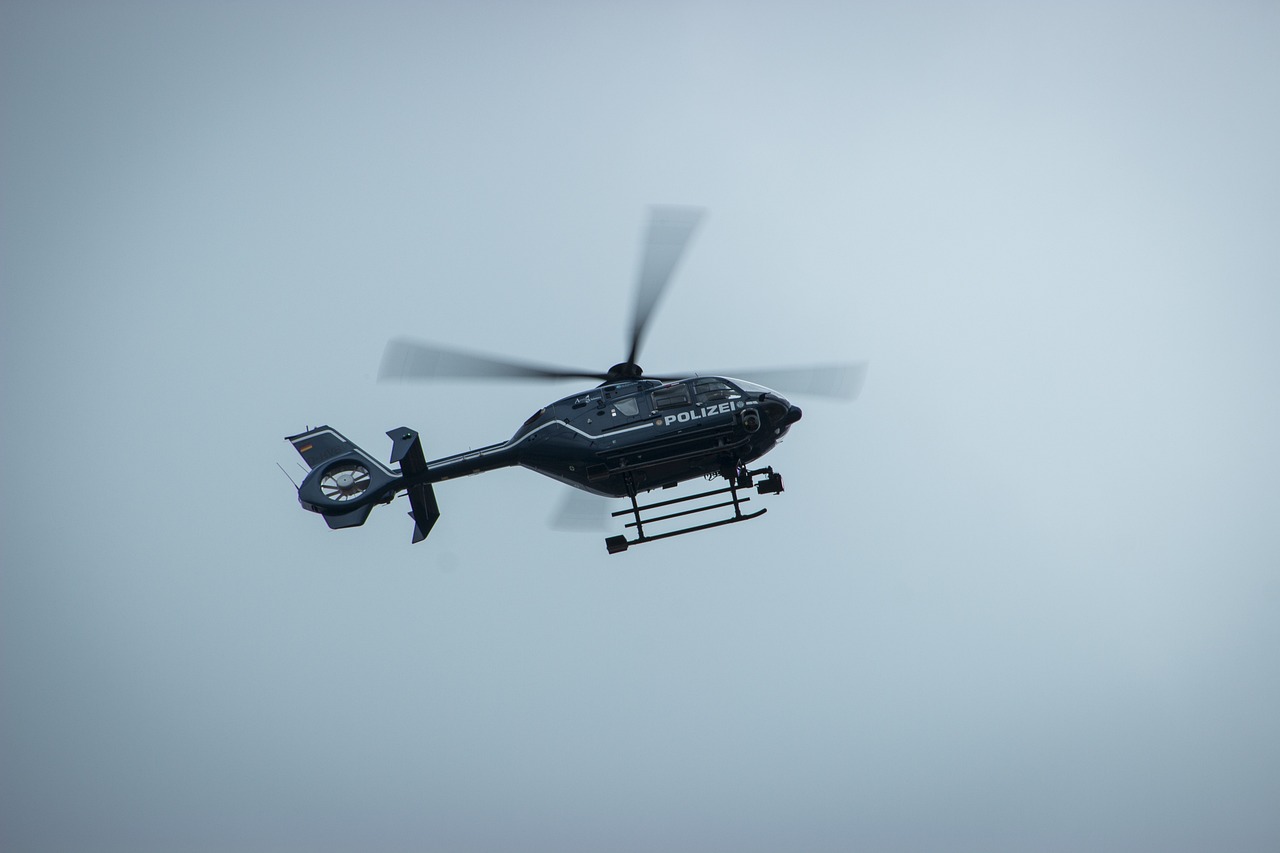
Predictive Analytics for Climate Change
Predictive analytics is becoming a game-changer in the fight against climate change. By utilizing sophisticated algorithms and vast datasets, researchers can model potential climate scenarios with remarkable accuracy. Imagine having a crystal ball that not only shows us the future but also helps us make informed decisions today. That's precisely what predictive analytics does—it allows us to foresee the impacts of climate change and devise strategies to mitigate them. With the power of artificial intelligence, we can analyze trends and patterns that were previously invisible, giving us a clearer picture of our environmental landscape.
One of the most exciting aspects of AI-driven predictive analytics is its ability to process enormous amounts of data from various sources. From satellite imagery to historical weather patterns, AI can crunch numbers and identify correlations that human analysts might miss. For instance, researchers can predict how rising temperatures will affect crop yields, water supply, and even the frequency of natural disasters. This predictive capability is not just about understanding what might happen; it's about preparing for it. Communities can develop adaptation strategies based on these insights, ensuring they are better equipped to handle the challenges ahead.
Consider this: if we know that a specific region is likely to experience more severe droughts in the coming years, local governments can implement water conservation measures or invest in drought-resistant crops. Similarly, predictive analytics can help us understand how coastal areas will respond to rising sea levels, prompting necessary infrastructure changes to protect vulnerable communities. It's a proactive approach, shifting the focus from reaction to prevention.
However, the journey of integrating predictive analytics into climate action isn't without its hurdles. One significant challenge is the quality of data. Inaccurate or incomplete data can lead to misleading predictions, which could have dire consequences. Therefore, it’s crucial to ensure that the data being fed into these AI systems is reliable and comprehensive. Moreover, as we rely more on AI, we must also address concerns related to algorithm bias. If the data reflects historical inequalities, the predictions may perpetuate those issues, leading to unfair outcomes for marginalized communities.
To illustrate the impact of predictive analytics, let’s take a look at a few case studies where AI has made a significant difference:
Case Study | Location | Outcome |
---|---|---|
Climate Impact Assessment | California | Improved water resource management |
Flood Risk Prediction | Bangladesh | Enhanced early warning systems |
Agricultural Yield Forecasting | India | Increased food security |
These examples highlight how predictive analytics can lead to tangible benefits, enabling societies to adapt and thrive in the face of climate change. The future of our planet may depend on how effectively we harness the power of AI and predictive analytics. By embracing these technologies, we can not only anticipate the challenges ahead but also create a sustainable future for generations to come.
As we look to the future, it’s essential to foster collaboration between scientists, policymakers, and technology developers. This interdisciplinary approach will ensure that predictive analytics evolves to meet the growing challenges posed by climate change. Together, we can unlock the full potential of AI to safeguard our environment and enhance our resilience against the inevitable changes that lie ahead.
- What is predictive analytics? Predictive analytics involves using statistical techniques and algorithms to analyze data and make predictions about future events.
- How does AI contribute to predictive analytics? AI enhances predictive analytics by processing large datasets quickly and identifying patterns that humans might overlook.
- What are the challenges of using predictive analytics for climate change? Challenges include data quality, algorithm bias, and the need for interdisciplinary collaboration.
- Can predictive analytics help in disaster preparedness? Yes, it can provide insights that help communities prepare for and respond to natural disasters more effectively.
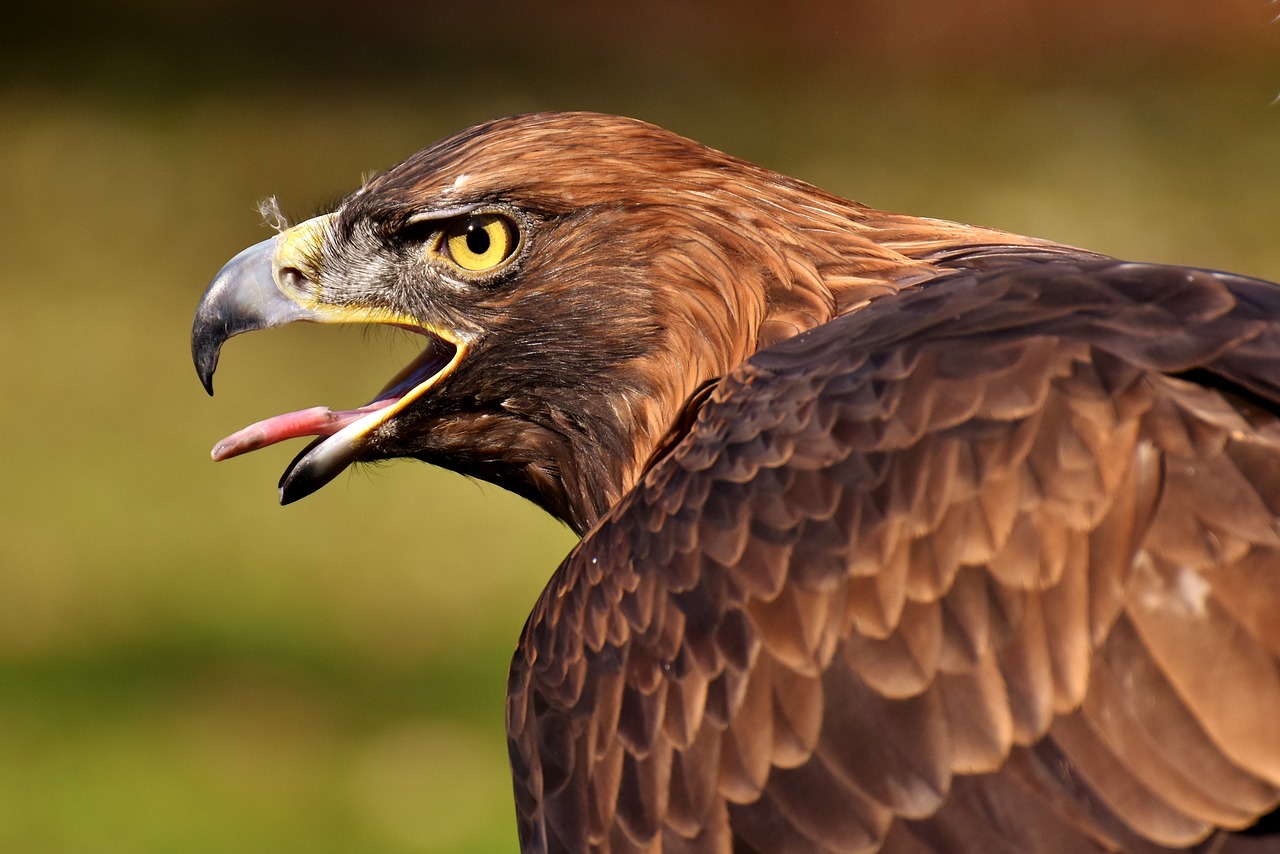
Wildlife Conservation and AI
In an age where wildlife conservation faces unprecedented challenges, the advent of artificial intelligence (AI) offers a beacon of hope. The integration of AI technologies into conservation efforts is not just a trend; it is a transformative approach that enhances our ability to monitor and protect endangered species. Imagine having the power to track animal movements, predict threats, and analyze population dynamics with unparalleled precision. This is the reality that AI brings to the table.
One of the most exciting applications of AI in wildlife conservation is through the use of camera traps combined with machine learning algorithms. These cameras, strategically placed in natural habitats, capture images of wildlife in their natural behavior. AI algorithms can analyze these images to identify species, count individuals, and even monitor their health. For instance, researchers have successfully deployed AI to monitor the population of the endangered snow leopard in the Himalayas. By analyzing thousands of images, AI can help conservationists understand the movement patterns and habitat use of these elusive animals, leading to more informed conservation strategies.
Moreover, AI is instrumental in combating poaching, a significant threat to wildlife. By employing predictive analytics, conservationists can identify areas at high risk for poaching activities. Machine learning models can analyze historical data, such as poaching incidents, weather patterns, and human activity, to forecast potential poaching events. This proactive approach allows rangers to allocate resources more effectively, increasing the chances of preventing illegal activities before they occur.
Additionally, AI can assist in habitat monitoring through the analysis of satellite imagery and drone footage. By processing vast amounts of data, AI can detect changes in land use, deforestation, and habitat degradation, which are critical for maintaining healthy ecosystems. For example, an AI system can analyze satellite images to track deforestation rates in the Amazon rainforest, providing vital information that can lead to conservation interventions.
However, the journey is not without its challenges. The successful implementation of AI in wildlife conservation requires collaboration among ecologists, data scientists, and local communities. Ensuring that AI systems are trained on diverse and representative datasets is crucial to avoid biases that could lead to ineffective conservation strategies. Furthermore, ethical considerations surrounding data privacy and the potential misuse of technology must be addressed to build trust among stakeholders.
In conclusion, the intersection of AI and wildlife conservation represents a promising frontier that can significantly enhance our efforts to protect endangered species and their habitats. As we harness the power of AI, we move closer to a future where technology and nature coexist harmoniously, paving the way for sustainable conservation practices that benefit both wildlife and humanity.
- How does AI help in wildlife conservation? AI assists in monitoring animal populations, predicting poaching threats, and analyzing habitat changes, enhancing conservation strategies.
- What are some examples of AI in wildlife tracking? AI-powered camera traps and satellite imagery analysis are commonly used to track and monitor wildlife.
- Are there any challenges in using AI for conservation? Yes, challenges include data privacy concerns, algorithm bias, and the need for interdisciplinary collaboration.
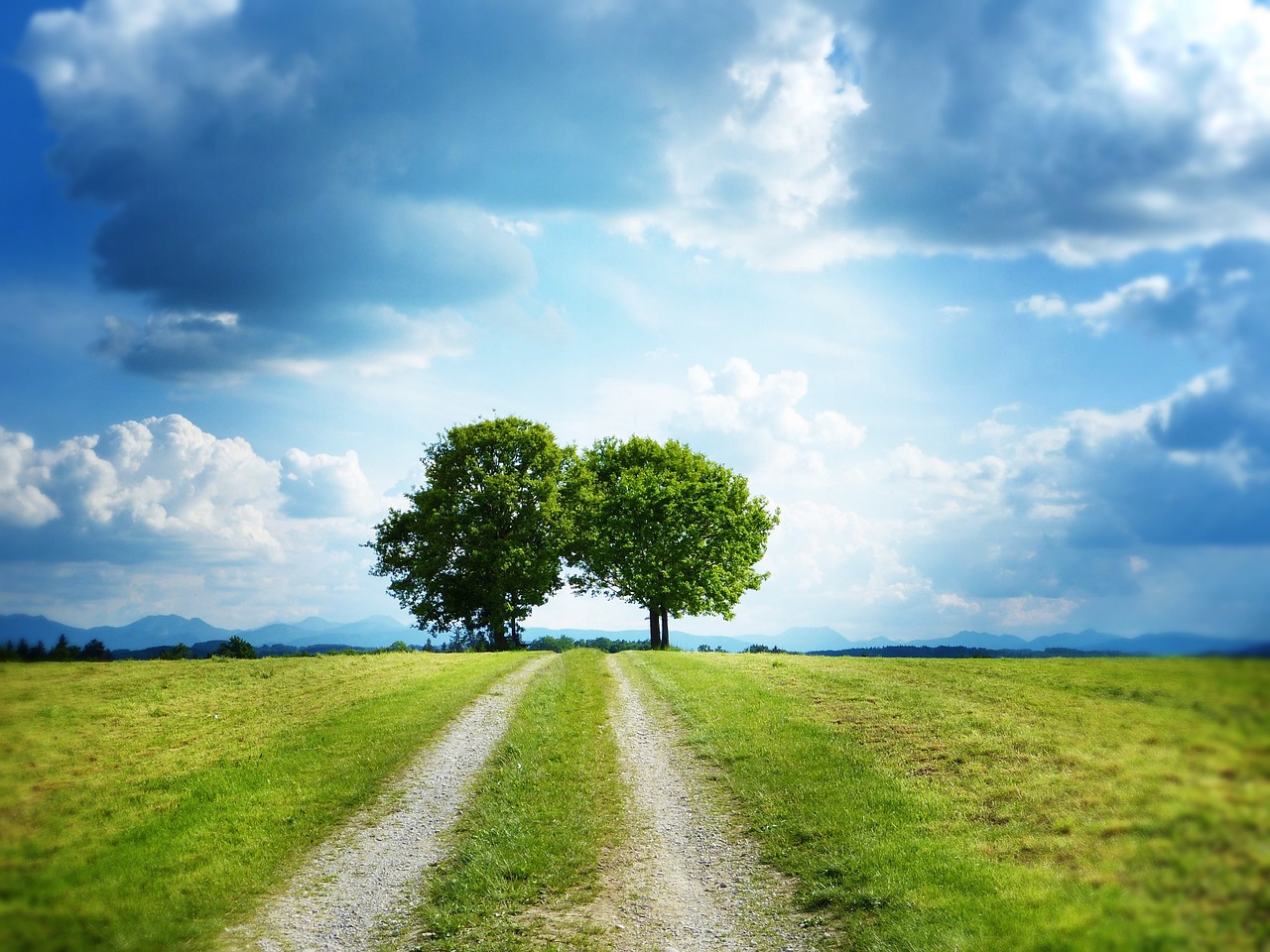
Remote Sensing and AI Integration
The integration of artificial intelligence (AI) with remote sensing technologies is a game-changer in the realm of environmental monitoring. Imagine having the ability to analyze vast swathes of data collected from satellites, drones, and other remote sensing devices in real-time. This powerful combination allows researchers and environmentalists to gain insights into land use, deforestation, urban expansion, and changes in natural habitats with unprecedented accuracy and speed. By leveraging AI algorithms, we can process and interpret the enormous datasets generated by remote sensing, turning raw data into actionable intelligence.
One of the most exciting aspects of this integration is the capability for predictive analytics. For instance, using machine learning models, we can predict how land cover will change over time based on current trends. This predictive power enables policymakers and conservationists to make informed decisions about land management and resource allocation. Consider this: if we can forecast where deforestation is likely to occur, we can implement protective measures before it's too late, thereby preserving vital ecosystems.
Moreover, AI enhances the accuracy of remote sensing data interpretation. Traditional methods of analyzing satellite images can be labor-intensive and prone to human error. However, AI can automate these processes, leading to faster and more reliable outcomes. For example, AI can identify patterns and anomalies in land use that might not be immediately visible to the naked eye. This capability is crucial for monitoring changes in biodiversity and detecting illegal activities such as poaching or illegal logging.
Benefits of AI and Remote Sensing Integration | Description |
---|---|
Real-Time Monitoring | AI enables instant analysis of remote sensing data, allowing for immediate responses to environmental changes. |
Enhanced Accuracy | Machine learning algorithms improve the precision of data interpretation, reducing human error. |
Predictive Capabilities | AI can forecast future environmental scenarios, aiding in proactive conservation efforts. |
Resource Optimization | Efficient allocation of resources based on data-driven insights maximizes conservation efforts. |
However, the integration of AI and remote sensing is not without its challenges. Data privacy is a significant concern, especially when dealing with sensitive information about land ownership or indigenous territories. Additionally, there is a need for interdisciplinary collaboration to ensure that the technology is used responsibly and effectively. Environmental scientists, data analysts, and policymakers must work together to create frameworks that guide the ethical use of AI in environmental monitoring.
In conclusion, the synergy between AI and remote sensing has the potential to revolutionize how we monitor and manage our planet's resources. As we continue to innovate and refine these technologies, we can look forward to a future where environmental sustainability is not just a goal but a reality. The possibilities are endless, and the implications for conservation and environmental health are profound.
- What is remote sensing? Remote sensing refers to the acquisition of information about an object or phenomenon without making physical contact, typically through satellite or aerial imagery.
- How does AI improve remote sensing? AI enhances remote sensing by automating data analysis, improving accuracy, and providing predictive insights for better decision-making.
- What are the main challenges of integrating AI with remote sensing? Key challenges include data privacy concerns, algorithm bias, and the need for interdisciplinary collaboration among experts.
- Can AI help in disaster management? Yes, AI can analyze remote sensing data to predict natural disasters, assess damage, and optimize response strategies.
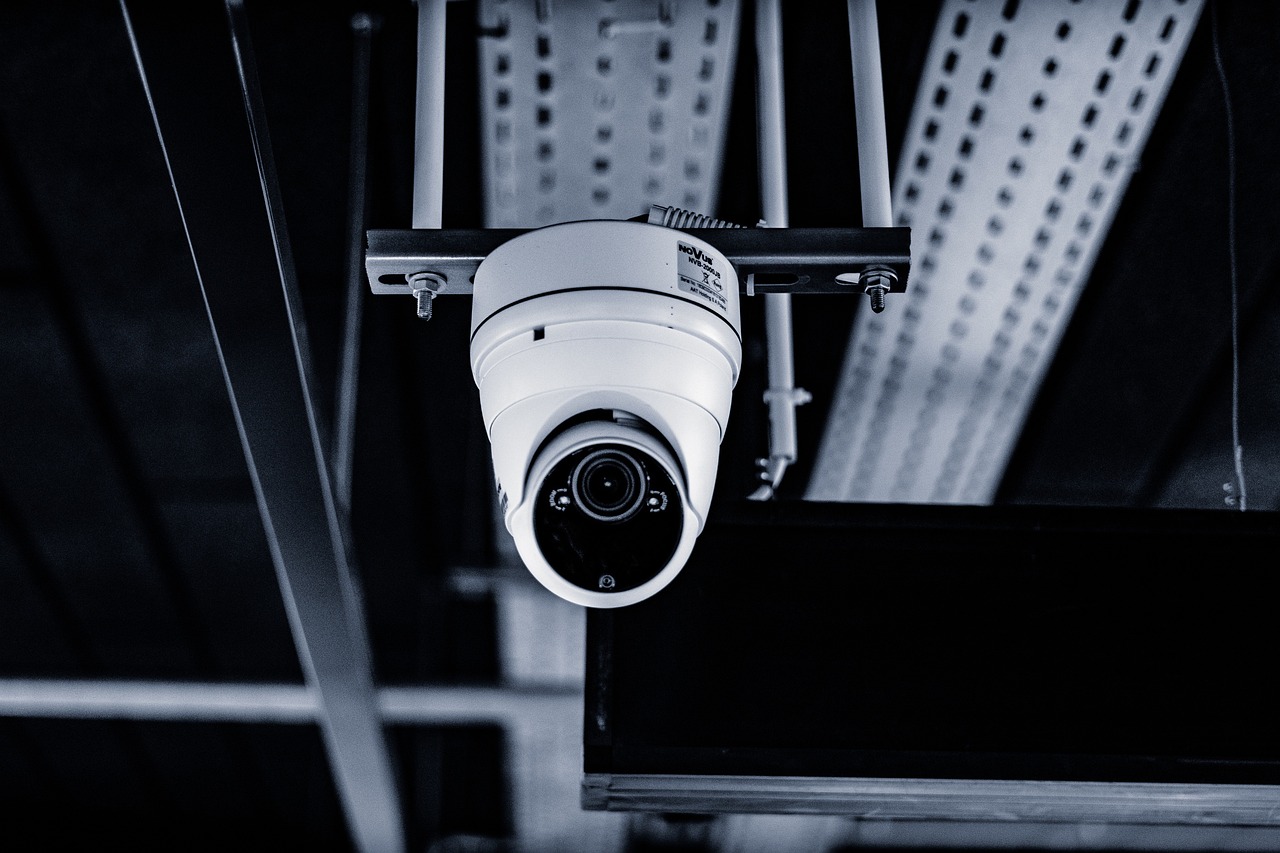
Challenges and Limitations of AI in Environmental Monitoring
While the integration of artificial intelligence (AI) into environmental monitoring brings a plethora of advantages, it is not without its challenges and limitations. One of the most pressing issues is data privacy. As AI systems often rely on large datasets, the collection and use of personal data can lead to significant privacy concerns. For instance, monitoring air quality in urban areas might necessitate the collection of data from various sensors, some of which may inadvertently capture personal information. This raises ethical questions about consent and the appropriate use of data.
Another major concern is the potential for algorithm bias. AI systems learn from historical data, and if that data is skewed or unrepresentative, the algorithms can perpetuate existing inequalities. For example, if air quality monitoring primarily focuses on affluent neighborhoods, the resulting predictions may overlook pollution hotspots in underprivileged areas. This not only hampers effective policy-making but can also exacerbate environmental injustices.
Moreover, the complexity of ecological systems poses a significant challenge for AI applications. Environmental data is often multifaceted and can be influenced by a myriad of factors, including climate, geography, and human activity. This complexity makes it difficult for AI models to accurately predict outcomes or identify trends without extensive training and refinement. Researchers must continuously update these models to ensure they remain relevant and effective, which can be resource-intensive.
Additionally, the need for interdisciplinary collaboration cannot be overstated. Environmental monitoring often requires expertise from various fields such as ecology, data science, and public policy. However, bridging the gap between these disciplines can be challenging. Effective communication and collaboration are essential for the successful implementation of AI solutions, yet many organizations struggle with these aspects, leading to fragmented efforts.
In summary, while AI holds great promise for enhancing environmental monitoring, it is crucial to address these challenges head-on. By prioritizing data privacy, mitigating algorithm bias, simplifying complex ecological data, and fostering interdisciplinary collaboration, we can harness the full potential of AI in our quest for a sustainable future.
- What are the main benefits of using AI in environmental monitoring?
AI enhances data analysis, predictive modeling, and real-time monitoring, leading to better decision-making and environmental protection. - How does algorithm bias affect environmental monitoring?
Algorithm bias can result in unfair or inaccurate predictions, particularly if the data used to train the AI is unrepresentative. - What steps can be taken to improve data privacy in AI applications?
Implementing strict data governance policies and ensuring transparency in data collection processes can help protect personal privacy. - Why is interdisciplinary collaboration important in AI environmental monitoring?
Different fields bring unique insights and expertise that are essential for effectively addressing complex environmental challenges.
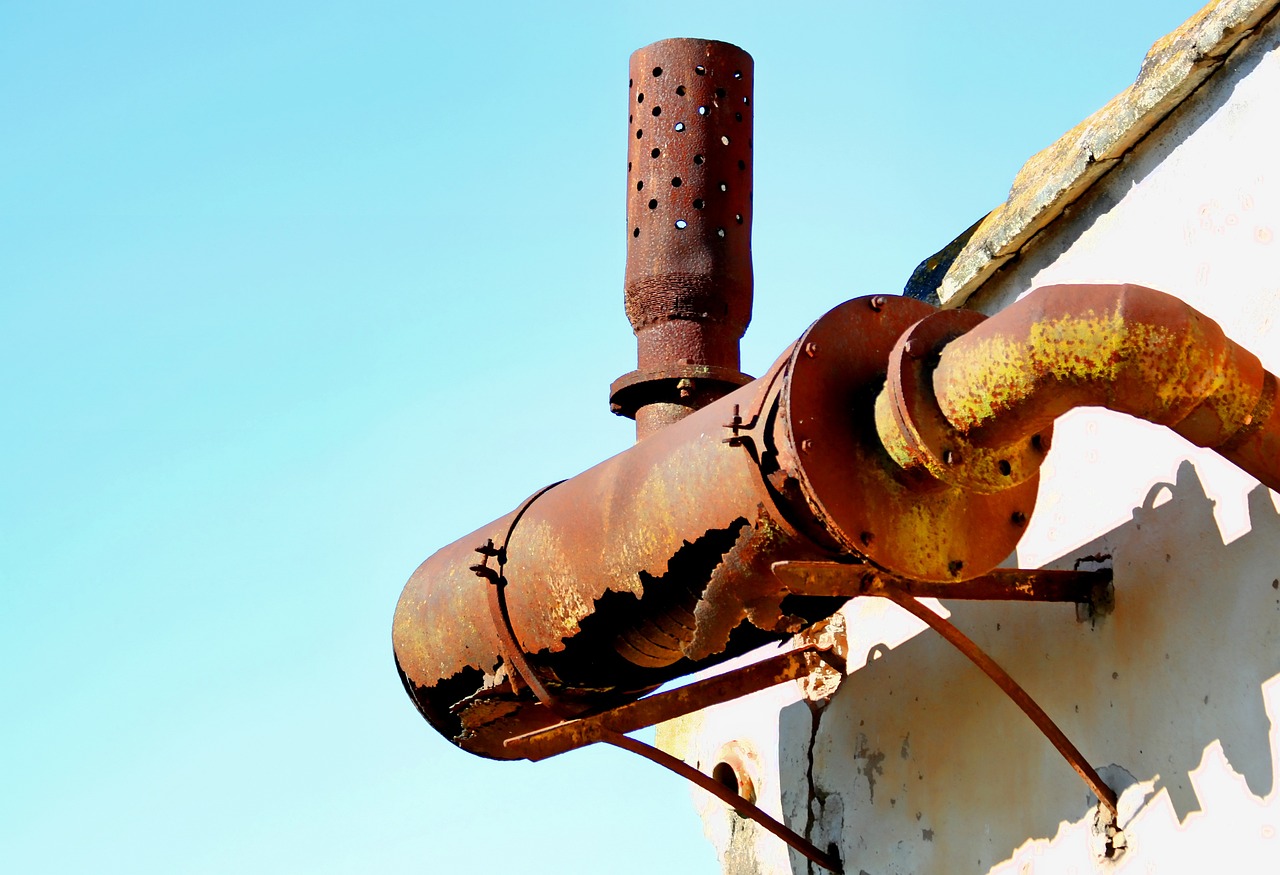
The Future of AI in Environmental Sustainability
The future of artificial intelligence (AI) in environmental sustainability is nothing short of exhilarating. As we stand on the brink of a technological revolution, the potential for AI to transform our approach to environmental challenges is immense. Imagine a world where we can predict environmental disasters before they happen, optimize resource use with pinpoint accuracy, and restore ecosystems with the help of intelligent algorithms. This is not just a dream; it is a tangible future that is rapidly unfolding.
One of the most promising aspects of AI in sustainability is its ability to process vast amounts of data in real-time. With the integration of AI technologies, we can collect and analyze data from various sources—ranging from satellite imagery to IoT sensors—allowing us to monitor environmental changes more effectively than ever before. For instance, AI can help in understanding complex ecosystems by identifying patterns and trends that human analysts might overlook. This capability is crucial for making informed decisions that can lead to sustainable practices.
Additionally, AI can drive innovation in renewable energy sectors. By optimizing energy consumption and predicting demand, AI systems can enhance the efficiency of solar panels and wind turbines, making them more viable alternatives to fossil fuels. This shift not only supports environmental sustainability but also fosters economic growth by creating new jobs in green technology. As we look to the future, the role of AI in energy management will likely become even more pronounced, leading to a significant reduction in carbon footprints globally.
Moreover, AI can facilitate better waste management practices. With machine learning algorithms, we can develop smarter recycling systems that automatically sort materials, reducing contamination rates and improving recycling efficiency. Imagine smart bins that can identify the type of waste and guide users on how to dispose of it properly. This kind of innovation not only enhances recycling rates but also educates the public about sustainable waste practices.
However, the journey towards a sustainable future with AI is not without its challenges. As we embrace these technologies, it is essential to address issues such as data privacy and algorithmic bias. Ensuring that AI systems are transparent and equitable will be critical in maintaining public trust and achieving widespread adoption. Furthermore, interdisciplinary collaboration among scientists, technologists, and policymakers will be vital to harness the full potential of AI in environmental sustainability.
In conclusion, the future of AI in environmental sustainability is bright and full of possibilities. As we continue to explore and innovate, we must remain vigilant about the ethical implications of these technologies. By leveraging AI responsibly, we can pave the way for a greener, more sustainable planet for generations to come.
- How can AI help in combating climate change?
AI can analyze large datasets to model climate scenarios, predict outcomes, and suggest mitigation strategies, helping to combat climate change effectively. - What role does AI play in wildlife conservation?
AI technologies are used to monitor animal populations, track movements, and identify threats, enhancing conservation efforts. - Are there any risks associated with using AI in environmental monitoring?
Yes, challenges include data privacy concerns, algorithm bias, and the need for interdisciplinary collaboration to ensure effective implementation.
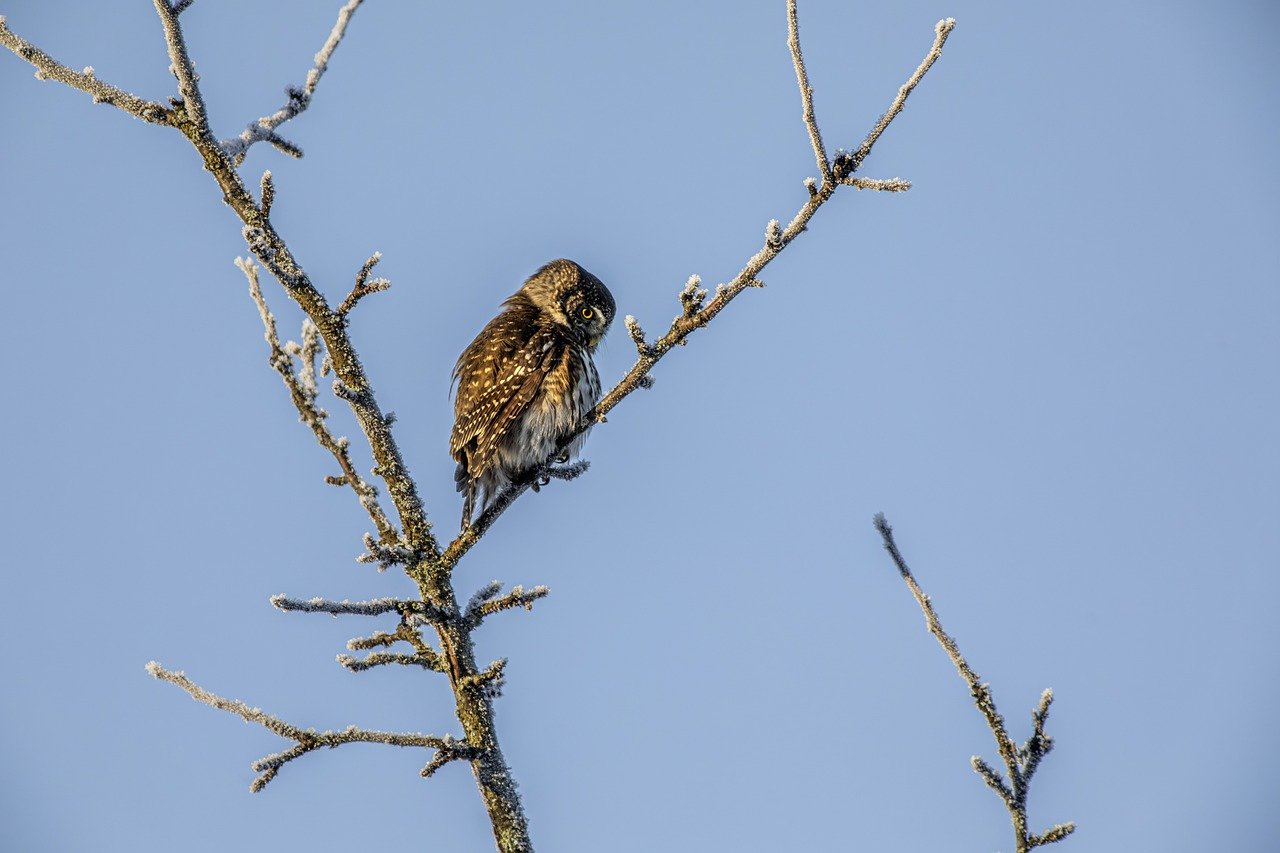
Case Studies: Successful AI Implementations
The integration of artificial intelligence in environmental monitoring has led to remarkable advancements, as evidenced by several successful case studies around the globe. These implementations not only highlight the potential of AI but also provide valuable insights into how technology can be harnessed to address pressing environmental issues. One notable example is the use of AI in air quality monitoring in cities like Los Angeles, where machine learning algorithms analyze data from various sensors to predict pollution levels. By identifying pollution hotspots, city planners can implement targeted interventions, leading to improved air quality and public health.
Another compelling case is found in the realm of water quality assessment. In the Great Lakes region, researchers have deployed AI to analyze sensor data from buoys that monitor water conditions. This technology not only detects the presence of harmful algal blooms but also predicts their spread, allowing for timely alerts to local communities and governments. The use of AI has significantly enhanced the speed and accuracy of water quality assessments, demonstrating its critical role in protecting aquatic ecosystems.
Moreover, the application of AI in wildlife conservation has shown promising results. In Africa, conservationists are utilizing AI-powered drones equipped with cameras to monitor animal populations in real-time. These drones can cover vast areas, tracking movements and detecting poaching activities with unmatched efficiency. This technology has already led to a decrease in poaching incidents and an increase in the population of endangered species, exemplifying how AI can be a game-changer in conservation efforts.
Furthermore, the integration of AI with remote sensing technologies has transformed land use management. A notable project in Brazil employed AI to analyze satellite imagery and assess deforestation rates in the Amazon rainforest. By processing large datasets quickly, AI has enabled researchers to identify illegal logging activities and promote sustainable land management practices. This proactive approach not only aids in conservation but also supports local communities reliant on forest resources.
Despite these successes, it’s essential to acknowledge the challenges faced in implementing AI solutions. Issues such as data privacy, algorithm bias, and the need for interdisciplinary collaboration can hinder progress. For instance, in urban areas, the collection of air quality data must balance public health interests with individual privacy rights. Additionally, ensuring that AI algorithms are free from bias is crucial to delivering equitable environmental solutions.
In summary, the case studies of AI implementations in environmental monitoring demonstrate not only the technology's potential but also the importance of collaboration among scientists, policymakers, and communities. As we move forward, these examples can serve as a blueprint for future projects aimed at enhancing our understanding and protection of the environment.
- What are some common applications of AI in environmental monitoring?
AI is commonly used in air quality assessment, water quality monitoring, wildlife conservation, and climate change predictive analytics. - How does AI improve the accuracy of environmental data?
AI algorithms can analyze vast amounts of data quickly and identify patterns that may not be visible to human analysts, leading to more accurate predictions and assessments. - Are there any risks associated with using AI in environmental monitoring?
Yes, risks include data privacy concerns, algorithm bias, and the need for interdisciplinary collaboration to ensure effective implementation. - Can AI help in combating climate change?
Absolutely! AI-driven predictive analytics can model climate change scenarios, helping researchers and policymakers develop effective strategies to mitigate its impacts.
Frequently Asked Questions
- What is the role of AI in environmental monitoring?
AI plays a transformative role in environmental monitoring by providing advanced tools for data analysis, predictive modeling, and real-time monitoring of ecological systems. This helps us better understand environmental changes and make informed decisions for sustainability.
- How does AI improve air quality monitoring?
AI algorithms analyze air quality data to predict pollution levels and identify sources of contaminants. This contributes to healthier urban environments and aids policymakers in creating effective regulations to combat air pollution.
- Can AI help in assessing water quality?
Absolutely! AI utilizes sensor data and machine learning to efficiently detect pollutants in water bodies, assessing the health of aquatic ecosystems and ensuring safe water for communities.
- What are predictive analytics in the context of climate change?
AI-driven predictive analytics model climate change scenarios, providing researchers with insights to prepare and respond to its impacts. This helps in developing strategies to mitigate adverse effects on ecosystems and human populations.
- How does AI contribute to wildlife conservation?
AI technologies monitor animal populations, track movements, and identify threats, which enhances efforts to protect endangered species and their habitats, ensuring a balanced ecosystem.
- What is the significance of remote sensing combined with AI?
The integration of AI with remote sensing technologies allows for the analysis of large volumes of environmental data, facilitating better land use management and resource allocation, which is crucial for sustainable development.
- What challenges does AI face in environmental monitoring?
Despite its advantages, AI in environmental monitoring faces challenges like data privacy concerns, algorithm bias, and the necessity for interdisciplinary collaboration to ensure effective solutions.
- What is the future of AI in environmental sustainability?
The future looks bright! AI holds immense potential for advancing environmental sustainability, driving innovations in monitoring practices, and supporting global efforts to combat climate change and biodiversity loss.
- Are there successful case studies of AI in environmental monitoring?
Yes, numerous case studies showcase successful AI implementations in environmental monitoring, highlighting tangible benefits and lessons learned that can guide future projects in this critical field.